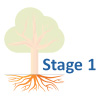
In the world of statistical analyses, there are many tests and methods that for categorical data. Many become extremely complex, especially as the number of variables increases. But sometimes we need an analysis for only one or two categorical variables at a time. When that is the case, one of these seven fundamental tests may come in handy.
These tests apply to nominal data (categories with no order to them) and a few can apply to other types of data as well. They allow us to test for goodness of fit, independence, or homogeneity—and yes, we will discuss the difference! Whether these tests are new to you, or you need a good refresher, this training will help you understand how they work and when each is appropriate to use.
(more…)
I was recently asked about when to use one and two tailed tests.
The long answer is: Use one tailed tests when you have a specific hypothesis about the direction of your relationship. Some examples include you hypothesize that one group mean is larger than the other; you hypothesize that the correlation is positive; you hypothesize that the proportion is below .5.
The short answer is: Never use one tailed tests.
Why?
1. Only a few statistical tests even can have one tail: z tests and t tests. So you’re severely limited. F tests, Chi-square tests, etc. can’t accommodate one-tailed tests because their distributions are not symmetric. Most statistical methods, such as regression and ANOVA, are based on these tests, so you will rarely have the chance to implement them.
2. Probably because they are rare, reviewers balk at one-tailed tests. They tend to assume that you are trying to artificially boost the power of your test. Theoretically, however, there is nothing wrong with them when the hypothesis and the statistical test are right for them.