When you have data measuring the time to an event, you can examine the relationship between various predictor variables and the time to the event using a Cox proportional hazards model.
In this webinar, you will see what a hazard function is and describe the interpretations of increasing, decreasing, and constant hazard. Then you will examine the log rank test, a simple test closely tied to the Kaplan-Meier curve, and the Cox proportional hazards model.
Note: This training is an exclusive benefit to members of the Statistically Speaking Membership Program and part of the Stat’s Amore Trainings Series. Each Stat’s Amore Training is approximately 90 minutes long.
(more…)
What is the relationship between predictors and whether and when an event will occur?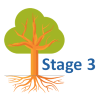
This is what event history (a.k.a., survival) analysis tests.
There are many flavors of Event History Analysis, though, depending on how time is measured, whether events can repeat, etc.
In this webinar, we discussed many of the issues involved in measuring time, including censoring, and introduce one specific type of event history model: the logistic model for discrete time events.
Note: This training is an exclusive benefit to members of the Statistically Speaking Membership Program and part of the Stat’s Amore Trainings Series. Each Stat’s Amore Training is approximately 90 minutes long.
(more…)
Need to dummy code in a Cox regression model?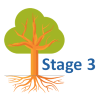
Interpret interactions in a logistic regression?
Add a quadratic term to a multilevel model?
This is where statistical analysis starts to feel really hard. You’re combining two difficult issues into one.
You’re dealing with both a complicated modeling technique at Stage 3 (survival analysis, logistic regression, multilevel modeling) and tricky effects in the model (dummy coding, interactions, and quadratic terms).
The only way to figure it all out in a situation like that is to break it down into parts. (more…)
Sure. One of the big advantages of multiple imputation is that you can use it for any analysis.
It’s one of the reasons big data libraries use it–no matter how researchers are using the data, the missing data is handled the same, and handled well.
I say this with two caveats. (more…)
Censored data are inherent in any analysis, like Event History or Survival Analysis, in which the outcome measures the Time to Event TTE. Censoring occurs when the event doesn’t occur for an observed individual during the time we observe them.
Despite the name, the event of “survival” could be any categorical event that you would like to describe the mean or median TTE. To take the censoring into account, though, you need to make sure your data are set up correctly.
Here is a simple example, for a data set that measures days after surgery until an (more…)
Time to event analyses (aka, Survival Analysis and Event History Analysis) are used often within medical, sales and epidemiological research. Some examples of time-to-event analysis are measuring the median time to death after being diagnosed with a heart condition, comparing male and female time to purchase after being given a coupon and estimating time to infection after exposure to a disease.
Survival time has two components that must be clearly defined: a beginning point and an endpoint that is reached either when the event occurs or when the follow-up time has ended.
One basic concept needed to understand time-to-event (TTE) analysis is censoring.
In simple TTE, you should have two types of observations:
1. The event occurred, and we are able to measure when it occurred OR
2. The event did NOT occur during the time we observed the individual, and we only know the total number of days in which it didn’t occur. (CENSORED).
Again you have two groups, one where the time-to-event is known exactly and one where it is not. The latter group is only known to have a certain amount of time where the event of interest did not occur. We don’t know if it would have occurred had we observed the individual longer. But knowing that it didn’t occur for so long tells us something about the risk of the envent for that person.
For example, let the time-to-event be a person’s age at onset of cancer. If you stop following someone after age 65, you may know that the person did NOT have cancer at age 65, but you do not have any information after that age.
You know that their age of getting cancer is greater than 65. But you do not know if they will never get cancer or if they’ll get it at age 66, only that they have a “survival” time greater than 65 years. They are censored because we did not gather information on that subject after age 65.
So one cause of censoring is merely that we can’t follow people forever. At some point you have to end your study, and not all people will have experienced the event.
But another common cause is that people are lost to follow-up during a study. This is called random censoring. It occurs when follow-up ends for reasons that are not under control of the investigator.
In survival analysis, censored observations contribute to the total number at risk up to the time that they ceased to be followed. One advantage here is that the length of time that an individual is followed does not have to be equal for everyone. All observations could have different amounts of follow-up time, and the analysis can take that into account.
Allison, P. D. (1995). Survival Analysis Using SAS. Cary, NC: SAS Institute Inc.
Hosmer, D. W. (2008). Applied Survival Analysis (2nd ed.). Hoboken, NJ: John Wiley & Sons, Inc.