Creating a quality scale for a latent construct (a variable that cannot be directly measured with one variable) takes many steps. Structural Equation Modeling is set up well for this task.
One important step in creating scales is making sure the scale measures the latent construct equally well and the same way for different groups of individuals.
(more…)
Whenever you use a multi-item scale to measure a construct, a key step is to create a score for each subject in the data set.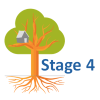
This score is an estimate of the value of the latent construct (factor) the scale is measuring for each subject. In fact, calculating this score is the final step of running a Confirmatory Factor Analysis.
(more…)
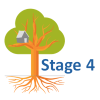
An extremely useful area of statistics is a set of models that use latent variables: variables whole values we can’t measure directly, but instead have to infer from others. These latent variables can be unknown groups, unknown numerical values, or unknown patterns in trajectories.
(more…)
Based on questions I’ve been asked by clients, most analysts prefer using the factor analysis procedures in their general statistical software to run a confirmatory factor analysis.
While this can work in some situations, you’re losing out on some key information you’d get from a structural equation model. This article highlights one of these.
(more…)
Confirmatory factor analysis (CFA) is the fundamental first step in running most types of SEM models. You want to do this first to verify the measurement quality of any and all latent constructs you’re using in your structural equation model.
(more…)
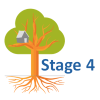
The last, and sometimes hardest, step for running any statistical model is writing up results.
As with most other steps, this one is a bit more complicated for structural equation models than it is for simpler models like linear regression.
Any good statistical report includes enough information that someone else could replicate your results with your data.
(more…)