In a previous post we discussed using marginal means to explain an interaction to a non-statistical audience. The output from a linear regression model can be a bit confusing. This is the model that was shown.
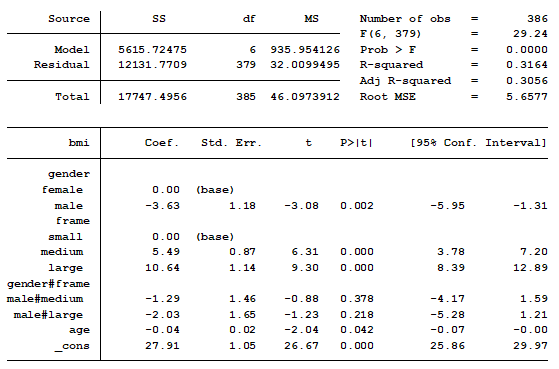
In this model, BMI is the outcome variable and there are three predictors:
(more…)
We’ve talked a lot around here about the reasons to use syntax — not only menus — in your statistical analyses.
Regardless of which software you use, the syntax file is pretty much always a text file. This is true for R, SPSS, SAS, Stata — just about all of them.
This is important because it means you can use an unlikely tool to help you code: Microsoft Word.
I know what you’re thinking. Word? Really?
Yep, it’s true. Essentially it’s because Word has much better Search-and-Replace options than your stat software’s editor.
Here are a couple features of Word’s search-and-replace that I use to help me code faster:
(more…)
In a previous post we discussed the difficulties of spotting meaningful information when we work with a large panel data set.
Observing the data collapsed into groups, such as quartiles or deciles, is one approach to tackling this challenging task. We showed how this can be easily done in Stata using just 10 lines of code.
As promised, we will now show you how to graph the collapsed data. (more…)
Panel data provides us with observations over several time periods per subject. In this first of two blog posts, I’ll walk you through the process. (Stick with me here. In Part 2, I’ll show you the graph, I promise.)
The challenge is that some of these data sets are massive. For example, if we’ve collected data on 100,000 individuals over 15 time periods, then that means we have 1.5 million cells of information.
So how can we look through this massive amount of data and observe trends over the time periods that we have tracked? (more…)
There are many steps to analyzing a dataset. One of the first steps is to create tables and graphs of your variables in order to understand what is behind the thousands of numbers on your screen. But the type of table and graph you create depends upon the type of variable you are looking at.
There certainly isn’t much point in running a frequency table for a continuous variable with hundreds of unique observations. Creating a boxplot to look for outliers doesn’t make much sense if the variable is categorical. Creating a histogram for a dummy variable would be senseless as well.
How should you start this process? Should you create a spreadsheet listing all the names of the variables and list what type of variable they are? Should you paste the names into a Word document?
In this free webinar with Stata expert Jeff Meyer, you will discover the code to quickly determine the type of every variable in a dataset. By simply pressing the execute button on a do-file you will observe Stata placing each variable in a group (the macro) based on the type of variable it is.
You will watch, through the use of loops, Stata create the proper table and graph for each type of variable in a matter of minutes and output the data into a pdf file for future viewing. You will also receive the code to recreate and practice what you’ve learned.
**
Title: Improving Your Productivity by Unlocking the Power of Stata’s Macros and Loops
Date: Thurs, May 26, 2016
Time: 1-2 pm EDT
Presenter: Jeff Meyer
This webinar has already taken place. Please sign up below to get access to the video recording.
Jeff Meyer is a statistical consultant with The Analysis Factor, a stats mentor for Statistically Speaking membership, and a workshop instructor. Read more about Jeff here.
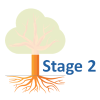
In a previous post , Using the Same Sample for Different Models in Stata, we examined how to use the same sample when comparing regression models. Using different samples in our models could lead to erroneous conclusions when interpreting results.
But excluding observations can also result in inaccurate results.
The coefficient for the variable “frequent religious attendance” was negative 58 in model 3 (more…)