When you put a continuous predictor into a linear regression model, you assume it has a constant relationship with the dependent variable along the predictor’s range. But how can you be certain? What is the best way to measure this?
And most important, what should you do if it clearly isn’t the case?
Let’s explore a few options for capturing a non-linear relationship between X and Y within a linear regression (yes, really). (more…)
Linear regression with a continuous predictor is set up to measure the constant relationship between that predictor and a continuous outcome.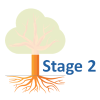
This relationship is measured in the expected change in the outcome for each one-unit change in the predictor.
One big assumption in this kind of model, though, is that this rate of change is the same for every value of the predictor. It’s an assumption we need to question, though, because it’s not a good approach for a lot of relationships.
Segmented regression allows you to generate different slopes and/or intercepts for different segments of values of the continuous predictor. This can provide you with a wealth of information that a non-segmented regression cannot.
In this webinar, we will cover (more…)