Most of the time when we plan a sample size for a data set, it’s based on obtaining reasonable statistical power for a key analysis of that data set. These power calculations figure out how big a sample you need so that a certain width of a confidence interval or p-value will coincide with a scientifically meaningful effect size.
But that’s not the only issue in sample size, and not every statistical analysis uses p-values.
(more…)
Ever hear this rule of thumb: “The Chi-Square test is invalid if we have fewer than 5 observations in a cell”.
I frequently hear this mis-understood and incorrect “rule.”
We all want rules of thumb even though we know they can be wrong, misleading, or misinterpreted.
Rules of Thumb are like Urban Myths or like a bad game of ‘Telephone’. The actual message gets totally distorted over time.
(more…)
The Kappa Statistic or Cohen’s* Kappa is a statistical measure of inter-rater reliability for categorical variables. In fact, it’s almost synonymous with inter-rater reliability.
Kappa is used when two raters both apply a criterion based on a tool to assess whether or not some condition occurs. Examples include:
(more…)
There are many rules of thumb in statistical analysis that make decision making and understanding results much easier.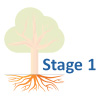
Have you ever stopped to wonder where these rules came from, let alone if there is any scientific basis for them? Is there logic behind these rules, or is it propagation of urban legends?
In this webinar, we’ll explore and question the origins, justifications, and some of the most common rules of thumb in statistical analysis, like:
(more…)