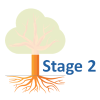
The last, and sometimes hardest, step for running any statistical model is writing up results.
As with most other steps, this one is a bit more complicated for structural equation models than it is for simpler models like linear regression.
Any good statistical report includes enough information that someone else could replicate your results with your data.
Today, I would like to briefly describe four misconceptions that I feel are commonly perceived by novice researchers in Exploratory Factor Analysis:
In Principal Component Analysis, a set of variables is transformed into a smaller set of linear composites known as components. This method of analysis is essentially a method for data reduction.
I recently gave a free webinar on Principal Component Analysis. We had almost 300 researchers attend and didn’t get through all the questions. This is part of a series of answers to those questions.
If you missed it, you can get the webinar recording here.
Answer:
Great question. Of course, the answer depends on your situation.
When you retain only one factor in a solution, then rotation is irrelevant. In fact, most software won’t even print out rotated coefficients and they’re pretty meaningless in that situation.
But if you retain two or more factors, you need to rotate.
Unrotated factors are pretty difficult to interpret in that situation. (more…)
I recently gave a free webinar on Principal Component Analysis. We had almost 300 researchers attend and didn’t get through all the questions. This is part of a series of answers to those questions.
If you missed it, you can get the webinar recording here.
Answer: Yes and no.
Principal Component Analysis specifically could be used with a training and test data set, but it doesn’t make as much sense as doing so for Factor Analysis.
That’s because PCA is really just about creating an index variable from a set of correlated predictors.
Factor Analysis is an actual model that is measuring a latent variable. Any time you’re creating some sort of scale to measure an underlying construct, you want to use Factor Analysis.
Factor Analysis is definitely best done with a training and test data set.
In fact, ideally, you’d run multiple rounds of training and test data sets, in which the variables included on your scale are updated after each test. (more…)
I recently gave a free webinar on Principal Component Analysis. We had almost 300 researchers attend and didn’t get through all the questions. This is part of a series of answers to those questions.
If you missed it, you can get the webinar recording here.
In fact, there were a few related but separate questions about using and interpreting the resulting component scores, so I’ll answer them together here.
Answer:
So yes, the point of PCA is to reduce variables — create an index score variable that is an optimally weighted combination of a group of correlated variables.
And yes, you can use this index variable as either a predictor or response variable.
It is often used as a solution for multicollinearity among predictor variables in a regression model. Rather than include multiple correlated predictors, none of which is significant, if you can combine them using PCA, then use that.
It’s also used as a solution to avoid inflated familywise Type I error caused by running the same analysis on multiple correlated outcome variables. Combine the correlated outcomes using PCA, then use that as the single outcome variable. (This is, incidentally, what MANOVA does).
In both cases, you can no longer interpret the individual variables.
You may want to, but you can’t. (more…)