What’s a good method for interpreting the results of a model with two continuous predictors and their interaction?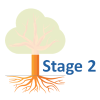
Let’s start by looking at a model without an interaction. In the model below, we regress a subject’s hip size on their weight and height. Height and weight are centered at their means.
(more…)
When a model has a binary outcome, one common effect size is a risk ratio. As a reminder, a risk ratio is simply a ratio of two probabilities. (The risk ratio is also called relative risk.)
Risk ratios are a bit trickier to interpret when they are less than one.
A predictor variable with a risk ratio of less than one is often labeled a “protective factor” (at least in Epidemiology). This can be confusing because in our typical understanding of those terms, it makes no sense that a risk be protective.
So how can a RISK be protective? (more…)
Predictor variables in statistical models can be treated as either continuous or categorical.
Usually, this is a very straightforward decision.
Categorical predictors, like treatment group, marital status, or highest educational degree should be specified as categorical.
Likewise, continuous predictors, like age, systolic blood pressure, or percentage of ground cover should be specified as continuous.
But there are numerical predictors that aren’t continuous. And these can sometimes make sense to treat as continuous and sometimes make sense as categorical.
(more…)
In your typical statistical work, chances are you have already used quantiles such as the median, 25th or 75th percentiles as descriptive statistics.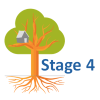
But did you know quantiles are also valuable in regression, where they can answer a broader set of research questions than standard linear regression?
In standard linear regression, the focus is on estimating the mean of a response variable given a set of predictor variables.
In quantile regression, we can go beyond the mean of the response variable. Instead we can understand how predictor variables predict (1) the entire distribution of the response variable or (2) one or more relevant features (e.g., center, spread, shape) of this distribution.
For example, quantile regression can help us understand not only how age predicts the mean or median income, but also how age predicts the 75th or 25th percentile of the income distribution.
Or we can see how the inter-quartile range — the width between the 75th and 25th percentile — is affected by age. Perhaps the range becomes wider as age increases, signaling that an increase in age is associated with an increase in income variability.
In this webinar, we will help you become familiar with the power and versatility of quantile regression by discussing topics such as:
- Quantiles – a brief review of their computation, interpretation and uses;
- Distinction between conditional and unconditional quantiles;
- Formulation and estimation of conditional quantile regression models;
- Interpretation of results produced by conditional quantile regression models;
- Graphical displays for visualizing the results of conditional quantile regression models;
- Inference and prediction for conditional quantile regression models;
- Software options for fitting quantile regression models.
Join us on this webinar to understand how quantile regression can be used to expand the scope of research questions you can address with your data.
Note: This training is an exclusive benefit to members of the Statistically Speaking Membership Program and part of the Stat’s Amore Trainings Series. Each Stat’s Amore Training is approximately 90 minutes long.
(more…)
In a simple linear regression model, how the constant (a.k.a., intercept) is interpreted depends upon the type of predictor (independent) variable.
If the predictor is categorical and dummy-coded, the constant is the mean value of the outcome variable for the reference category only. If the predictor variable is continuous, the constant equals the predicted value of the outcome variable when the predictor variable equals zero.
Removing the Constant When the Predictor Is Categorical
When your predictor variable X is categorical, the results are logical. Let’s look at an example. (more…)