Structural Equation Modeling (SEM) is a popular method to test hypothetical relationships between constructs in the social sciences. These constructs may be unobserved (a.k.a., “latent”) or
observed (a.k.a., “manifest”).
In this training, you will learn the different types of SEM: confirmatory factor analysis, path analysis for manifest and latent variables, and latent growth modeling (i.e., the application of SEM on longitudinal data).
We’ll discuss the different terminology, the commonly used symbols, and the different ways a model can be specified, as well as how to present results and evaluate the fit of the models.
This training will be at a very basic conceptual level; however, it is assumed that participants have an understanding of multiple regression, interpretation of statistical tests, and methods of data screening.
Note: This training is an exclusive benefit to members of the Statistically Speaking Membership Program and part of the Stat’s Amore Trainings Series. Each Stat’s Amore Training is approximately 90 minutes long.
(more…)
A multiple regression model could be conceptualized using Structural Equation Model path diagrams. That’s the simplest SEM you can create, but its real power lies in expanding on that regression model. Here I will discuss four types of structural equation models.
Path Analysis
More interesting research questions could be asked and answered using Path Analysis. Path Analysis is a type of structural equation modeling without latent variables. (more…)
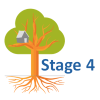
We get many questions from clients who use the terms mediator and moderator interchangeably.
They are easy to confuse, yet mediation and moderation are two distinct terms that require distinct statistical approaches.
The key difference between the concepts can be compared to a case where a moderator lets you know when an association will occur while a mediator will inform you how or why it occurs.
(more…)
Often a model is not a simple process from a treatment or intervention to the outcome. In essence, the value of X does not always directly predict the value of Y.
Mediators can affect the relationship between X and Y. Moderators can affect the scale and magnitude of that relationship. And sometimes the mediators and moderators affect each other.
One of the most confusing things about statistical analysis is the different vocabulary used for the same, or nearly-but-not-quite-the-same, concepts.
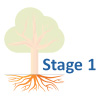
Sometimes this happens just because the same analysis was developed separately within different fields and named twice.
So people in different fields use different terms for the same statistical concept. Try to collaborate with a colleague in a different field and you may find yourself awed by the crazy statistics they’re insisting on.
Other times, there is a level of detail that is implied by one term that isn’t true of the wider, more generic term. This level of detail is often about how the role of variables or effects affects the interpretation of output. (more…)