I recently received a great question in a comment about whether the assumptions of normality, constant variance, and independence in linear models are about the errors, εi, or the response variable, Yi.
The asker had a situation where Y, the response, was not normally distributed, but the residuals were.
Quick Answer: It’s just the errors.
In fact, if you look at any (good) statistics textbook on linear models, you’ll see below the model, stating the assumptions: (more…)
The normal distribution is so ubiquitous in statistics that those of us who use a lot of statistics tend to forget it’s not always so common in actual data.
And since the normal distribution is continuous, many people describe all numerical variables as continuous. I get it: I’m guilty of using those terms interchangeably, too, but they’re not exactly the same.
Numerical variables can be either continuous or discrete.
The difference? Continuous variables can take any number within a range. Discrete variables can only be whole numbers.
So 3.04873658 is a possible value of a continuous variable, but not discrete.
Count variables, as the name implies, are frequencies of some event or state. Number of arrests, fish (more…)
A normal probability plot is extremely useful for testing normality assumptions. It’s more precise than a histogram, which can’t pick up subtle deviations, and doesn’t suffer from too much or too little power, as do tests of normality.
There are two versions of normal probability plots: Q-Q and P-P. I’ll start with the Q-Q. (more…)
The assumptions of normality and constant variance in a linear model (both OLS regression and ANOVA) are quite robust to departures. That means that even if the assumptions aren’t met perfectly, the resulting p-values will still be reasonable estimates.
But you need to check the assumptions anyway, because some departures are so far off that the p-values become inaccurate. And in many cases there are remedial measures you can take to turn non-normal residuals into normal ones.
But sometimes you can’t.
Sometimes it’s because the dependent variable just isn’t appropriate for a linear model. The (more…)
Here’s a little reminder for those of you checking assumptions in regression and ANOVA:
The assumptions of normality and homogeneity of variance for linear models are not about Y, the dependent variable. (If you think I’m either stupid, crazy, or just plain nit-picking, read on. This distinction really is important). (more…)
I often hear concern about the non-normal distributions of independent variables in regression models, and I am here to ease your mind.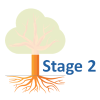
There are NO assumptions in any linear model about the distribution of the independent variables. Yes, you only get meaningful parameter estimates from nominal (unordered categories) or numerical (continuous or discrete) independent variables. But no, the model makes no assumptions about them. They do not need to be normally distributed or continuous.
It is useful, however, to understand the distribution of predictor variables to find influential outliers or concentrated values. A highly skewed independent variable may be made more symmetric with a transformation.