SPSS has a nice little feature for adding and averaging variables with
missing data that many people don’t know about.
It allows you to add or average variables that have some missing data, while specifying how many are allowed to be missing. (more…)
Ever consider skipping the important step of cleaning your data? It’s tempting but not a good idea. Why? It’s a bit like baking.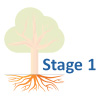
I like to bake. There’s nothing nicer than a rainy Sunday with no plans, and a pantry full of supplies. I have done my shopping, and now it’s time to make the cake. Ah, but the kitchen is a mess. I don’t have things in order. This is no way to start.
First, I need to clear the counter, wash the breakfast dishes, and set out my tools. I need to take stock, read the recipe, and measure out my ingredients. Then it’s time for the fun part. I’ll admit, in my rush to get started I have at times skipped this step.
(more…)
There’s no mincing words here. Missing values can cause problems for every statistician. That’s true for a lot of reasons, but it can start with simple issues of choices
made when coding missing values in a data set. Here are a few examples.
Example 1: The Null License Plate
Researcher Joseph Tartaro thought it would be funny to get the following California vanity license plate: (more…)
One of the important issues with missing data is the missing data mechanism. You may have heard of these: Missing Completely at Random (MCAR), Missing at Random (MAR), and Missing Not at Random (MNAR).
The mechanism is important because it affects how much the missing data bias your results. This has a big impact on what is a reasonable approach to dealing with the missing data. So you have to take it into account in choosing an approach.
The concepts of these mechanisms can be a bit abstract.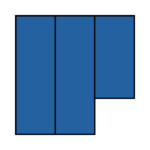
And to top it off, two of these mechanisms have really confusing names: Missing Completely at Random and Missing at Random.
Missing Completely at Random (MCAR)
Missing Completely at Random is pretty straightforward. What it means is what is (more…)
If you’ve been doing data analysis for long, you’ve probably had the ‘AHA’ moment where you realized statistical practice is a craft and not just a science. As with any craft, there are best practices that will save you a
lot of pain and suffering and elevate the quality of your work. And yet, it’s likely that no one may have taught you these. I know I never had a class on this. (more…)
Imputation as an approach to missing data has been around for decades.
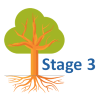
You probably learned about mean imputation in methods classes, only to be told to never do it for a variety of very good reasons. Mean imputation, in which each missing value is replaced, or imputed, with the mean of observed values of that variable, is not the only type of imputation, however. (more…)