Repeated measures ANOVA is the approach most of us learned in stats classes for repeated measures and longitudinal data. It works very well in certain designs.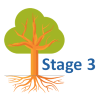
But it’s limited in what it can do. Sometimes trying to fit a data set into a repeated measures ANOVA requires too much data gymnastics. (more…)
“Because mixed models are more complex and more flexible than the general linear model, the potential for confusion and errors is higher.”
– Hamer & Simpson (2005)
Linear Mixed Models, as implemented in SAS’s Proc Mixed, SPSS Mixed, R’s LMER, and Stata’s xtmixed, are an extension of the general linear model. They use more sophisticated techniques for estimation of parameters (means, variances, regression coefficients, and standard errors), and as the quotation says, are much more flexible.
Here’s one example of the flexibility of mixed models, and its resulting potential for confusion and error. (more…)
There are three main ways you can approach analyzing repeated measures data, assuming the dependent variable is measured
continuously: repeated measures ANOVA, Mixed Models, and Marginal Models. Let’s take a look at how the three approaches differ and some of their advantages and disadvantages.
For a few, very specific designs, you can get the exact same results from all three approaches. This makes it difficult to figure out what each one is doing, and how to apply them to OTHER designs.
For the sake of the current discussion, I will define repeated measures data as repeated measurements of the same outcome variable on the same individual. The individual is often a person, but could just as easily be a plant, animal, colony, company, etc. For simplicity, I’ll use “individual.” (more…)