Ordinary Least Squares regression provides linear models of continuous variables. However, much data of interest to statisticians and researchers are not continuous and so other methods must be used to create useful predictive models.
The glm() command is designed to perform generalized linear models (regressions) on binary outcome data, count data, probability data, proportion data and many other data types.
In this blog post, we explore the use of R’s glm() command on one such data type. Let’s take a look at a simple example where we model binary data.
(more…)
Need to run a logistic regression in SPSS? Turns out, SPSS has a number of procedures for running different types of logistic regression.
Some types of logistic regression can be run in more than one procedure. For some unknown reason, some procedures produce output others don’t. So it’s helpful to be able to use more than one.
Logistic Regression
Logistic Regression can be used only for binary dependent (more…)
What is the relationship between predictors and whether and when an event will occur?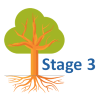
This is what event history (a.k.a., survival) analysis tests.
There are many flavors of Event History Analysis, though, depending on how time is measured, whether events can repeat, etc.
In this webinar, we discussed many of the issues involved in measuring time, including censoring, and introduce one specific type of event history model: the logistic model for discrete time events.
Note: This training is an exclusive benefit to members of the Statistically Speaking Membership Program and part of the Stat’s Amore Trainings Series. Each Stat’s Amore Training is approximately 90 minutes long.
(more…)
One great thing about logistic regression, at least for those of us who are trying to learn how to use it, is that the predictor variables work exactly the same way as they do in linear regression.
Dummy coding, interactions, quadratic terms–they all work the same way.
Dummy Coding
In pretty much every regression procedure in every stat software, the default way to code categorical variables is with dummy coding.
All dummy coding means is recoding the original categorical variable into a set of binary variables that have values of one and zero. You may find it helpful to (more…)
Graphing predicted values from a regression model or means from an ANOVA makes interpretation of results much easier.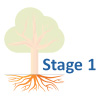
Every statistical software will graph predicted values for you. But the more complicated your model, the harder it can be to get the graph you want in the format you want.
Excel isn’t all that useful for estimating the statistics, but it has some very nice features that are useful for doing data analysis, one of which is graphing.
In this webinar, I will demonstrate how to calculate predicted means from a linear and a logistic regression model, then graph them. It will be particularly useful to you if you don’t have a very clear sense of where those predicted values come from.
Note: This training is an exclusive benefit to members of the Statistically Speaking Membership Program and part of the Stat’s Amore Trainings Series. Each Stat’s Amore Training is approximately 90 minutes long.
About the Instructor
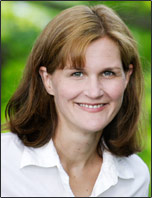
Karen Grace-Martin helps statistics practitioners gain an intuitive understanding of how statistics is applied to real data in research studies.
She has guided and trained researchers through their statistical analysis for over 15 years as a statistical consultant at Cornell University and through The Analysis Factor. She has master’s degrees in both applied statistics and social psychology and is an expert in SPSS and SAS.
Not a Member Yet?
It’s never too early to set yourself up for successful analysis with support and training from expert statisticians.
Just head over and sign up for Statistically Speaking.
You'll get access to this training webinar, 130+ other stats trainings, a pathway to work through the trainings that you need — plus the expert guidance you need to build statistical skill with live Q&A sessions and an ask-a-mentor forum.
Hierarchical regression is a very common approach to model building that allows you to see the incremental contribution to a model of sets of predictor variables.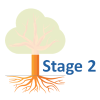
Popular for linear regression in many fields, the approach can be used in any type of regression model — logistic regression, linear mixed models, or even ANOVA.
In this webinar, we’ll go over the concepts and steps, and we’ll look at how it can be useful in different contexts.
Note: This training is an exclusive benefit to members of the Statistically Speaking Membership Program and part of the Stat’s Amore Trainings Series. Each Stat’s Amore Training is approximately 90 minutes long.
About the Instructor
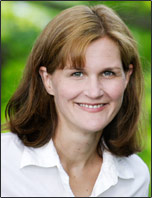
Karen Grace-Martin helps statistics practitioners gain an intuitive understanding of how statistics is applied to real data in research studies.
She has guided and trained researchers through their statistical analysis for over 15 years as a statistical consultant at Cornell University and through The Analysis Factor. She has master’s degrees in both applied statistics and social psychology and is an expert in SPSS and SAS.
Not a Member Yet?
It’s never too early to set yourself up for successful analysis with support and training from expert statisticians.
Just head over and sign up for Statistically Speaking.
You'll get access to this training webinar, 130+ other stats trainings, a pathway to work through the trainings that you need — plus the expert guidance you need to build statistical skill with live Q&A sessions and an ask-a-mentor forum.