December 20th, 2017 by Jeff Meyer
Sometimes what is most tricky about understanding your regression output is knowing exactly what your software is presenting to you.
Here’s a great example of what looks like two completely different model results from SPSS and Stata that in reality, agree.
The Model
I ran a linear model regressing “physical composite score” on education and “mental composite score”.
The outcome variable, physical composite score, is a measurement of one’s physical well-being. The predictor “education” is categorical with four categories. The other predictor, mental composite score, is continuous and measures one’s mental well-being.
I am interested in determining whether the association between physical composite score and mental composite score is different among the four levels of education. To determine this I included an interaction between mental composite score and education.
The SPSS Regression Output
Here is the result of the regression using SPSS:
(more…)
January 1st, 2014 by Karen Grace-Martin
In this follow-up to December’s webinar, we’ll finish up our discussion of interactions.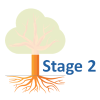
There is something about interactions that is incredibly confusing.
An interaction between two predictor variables means that one predictor variable affects a third variable differently at different values of the other predictor.
How you understand that interaction depends on many things, including:
- Whether one, or both, of the predictor variables is categorical or numerical
- How each of those variables is coded (specifically, whether each categorical variable is dummy or effect coded and whether numerical variables are centered)
- Whether it’s a two-way or three-way interaction
- Whether there is a directionality to the interaction (moderation) or not
Sometimes you need to get pretty sophisticated in your coding, in the output you ask for, and in writing out regression equations.
In this webinar, we’ll examine how to put together and break apart output to understand what your interaction is telling you.
Note: This training is an exclusive benefit to members of the Statistically Speaking Membership Program and part of the Stat’s Amore Trainings Series. Each Stat’s Amore Training is approximately 90 minutes long.
About the Instructor
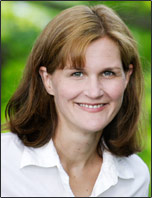
Karen Grace-Martin helps statistics practitioners gain an intuitive understanding of how statistics is applied to real data in research studies.
She has guided and trained researchers through their statistical analysis for over 15 years as a statistical consultant at Cornell University and through The Analysis Factor. She has master’s degrees in both applied statistics and social psychology and is an expert in SPSS and SAS.
Not a Member Yet?
It’s never too early to set yourself up for successful analysis with support and training from expert statisticians.
Just head over and sign up for Statistically Speaking.
You'll get access to this training webinar, 130+ other stats trainings, a pathway to work through the trainings that you need — plus the expert guidance you need to build statistical skill with live Q&A sessions and an ask-a-mentor forum.
December 1st, 2013 by Karen Grace-Martin
There is something about interactions that is incredibly confusing.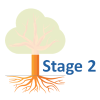
An interaction between two predictor variables means that one predictor variable affects a third variable differently at different values of the other predictor.
How you understand that interaction depends on many things, including:
- Whether one, or both, of the predictor variables is categorical or numerical
- How each of those variables is coded (specifically, whether each categorical variable is dummy or effect coded and whether numerical variables are centered)
- Whether it’s a two-way or three-way interaction
- Whether there is a directionality to the interaction (moderation) or not
Sometimes you need to get pretty sophisticated in your coding, in the output you ask for, and in writing out regression equations.
In this webinar, we’ll examine how to put together and break apart output to understand what your interaction is telling you.
Note: This training is an exclusive benefit to members of the Statistically Speaking Membership Program and part of the Stat’s Amore Trainings Series. Each Stat’s Amore Training is approximately 90 minutes long.
About the Instructor
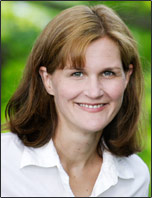
Karen Grace-Martin helps statistics practitioners gain an intuitive understanding of how statistics is applied to real data in research studies.
She has guided and trained researchers through their statistical analysis for over 15 years as a statistical consultant at Cornell University and through The Analysis Factor. She has master’s degrees in both applied statistics and social psychology and is an expert in SPSS and SAS.
Not a Member Yet?
It’s never too early to set yourself up for successful analysis with support and training from expert statisticians.
Just head over and sign up for Statistically Speaking.
You'll get access to this training webinar, 130+ other stats trainings, a pathway to work through the trainings that you need — plus the expert guidance you need to build statistical skill with live Q&A sessions and an ask-a-mentor forum.
May 17th, 2010 by Karen Grace-Martin
In a previous post, Interpreting Interactions in Regression, I said the following:
In our example, once we add the interaction term, our model looks like:
Height = 35 + 4.2*Bacteria + 9*Sun + 3.2*Bacteria*Sun
Adding the interaction term changed the values of B1 and B2. The effect of Bacteria on Height is now 4.2 + 3.2*Sun. For plants in partial sun, Sun = 0, so the effect of Bacteria is 4.2 + 3.2*0 = 4.2. So for two plants in partial sun, a plant with 1000 more bacteria/ml in the soil would be expected to be 4.2 cm taller than a (more…)
May 11th, 2009 by Karen Grace-Martin
The way to follow up on a significant two-way interaction between two categorical variables is to check the simple effects. Most of the time the simple effects tests give a very clear picture about the interaction. Every so often, however, you have a significant interaction, but no significant simple effects. It is not a logical impossibility. They are testing two different, but related hypotheses.
Assume your two independent variables are A and B. Each has two values: 1 and 2. The interaction is testing if A1 – B1 = A2 – B2 (the null hypothesis). The simple effects are testing whether A1-B1=0 and A2-B2=0 (null) or not.
If you have a crossover interaction, you can have A1-B1 slightly positive and A2-B2 slightly negative. While neither is significantly different from 0, they are significantly different from each other.
And it is highly useful for answering many research questions to know if the differences in the means in one condition equal the differences in the means for the other. It might be true that it’s not testing a hypothesis you’re interested in, but in many studies, all the interesting effects are in the interactions.
February 23rd, 2009 by Karen Grace-Martin
A Linear Regression Model with an interaction between two predictors (X1 and X2) has the form: 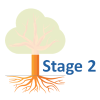
Y = B0 + B1X1 + B2X2 + B3X1*X2.
It doesn’t really matter if X1 and X2 are categorical or continuous, but let’s assume they are continuous for simplicity.
One important concept is that B1 and B2 are not main effects, the way they would be if (more…)