Sample size estimates are one of those data analysis tasks that look straightforward, but once you try to do one, make you want to bang your head against the computer in frustration. Or, maybe
that’s just me.
Regardless of how they make you feel, they are super important to do for your study before you collect the data.
(more…)
Here’s a common situation.
Your grant application or committee requires sample size estimates. It’s not the calculations that are hard (though they can be), it’s getting the information to fill into the calculations.
Every article you read on it says you need to either use pilot data or another similar study as a basis for the values to enter into the software.
You have neither.
No similar studies have ever used the scale you’re using for the dependent variable.
And while you’d love to run a pilot study, it’s just not possible. There are too many practical constraints — time, money, distance, ethics.
What do you do?
(more…)
There are many rules of thumb in statistical analysis that make decision making and understanding results much easier.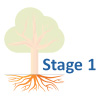
Have you ever stopped to wonder where these rules came from, let alone if there is any scientific basis for them? Is there logic behind these rules, or is it propagation of urban legends?
In this webinar, we’ll explore and question the origins, justifications, and some of the most common rules of thumb in statistical analysis, like:
(more…)
Whenever we run an analysis of variance or run a regression one of the first things we do is look at the p-value of our predictor variables to determine whether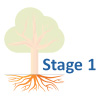
they are statistically significant. When the variable is statistically significant, did you ever stop and ask yourself how significant it is? (more…)
Effect size statistics are expected by many journal editors these days.
If you’re running an ANOVA, t-test, or linear regression model, it’s pretty straightforward which ones to report.
Things get trickier, though, once you venture into other types of models.
(more…)
R² is such a lovely statistic, isn’t it? Unlike so many of the others, it makes sense–the percentage of variance in Y accounted for by a model.
I mean, you can actually understand that. So can your grandmother. And the clinical audience you’re writing the report for.
A big R² is always good and a small one is always bad, right?
Well, maybe. (more…)