Effect size statistics are all the rage these days.
Journal editors are demanding them. Committees won’t pass dissertations without them.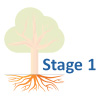
But the reason to compute them is not just that someone wants them — they can truly help you understand your data analysis.
What Is an Effect Size Statistic?
And yes, these definitely qualify. But the concept of an effect size statistic is actually much broader. Here’s a description from a nice article on effect size statistics:
If you think about it, many familiar statistics fit this description. Regression coefficients give information about the magnitude and direction of the relationship between two variables. So do correlation coefficients. (more…)
Effect size statistics are extremely important for interpreting statistical results. The emphasis on reporting them has been a great development over the past decade. (more…)
Lest you believe that odds ratios are merely the domain of logistic regression, I’m here to tell you it’s not true.
One of the simplest ways to calculate an odds ratio is from a cross tabulation table.
We usually analyze these tables with a categorical statistical test. There are a few options, depending on the sample size and the design, but common ones are Chi-Square test of independence or homogeneity, or a Fisher’s exact test.
(more…)
Effect size statistics are required by most journals and committees these days — for good reason.
They communicate just how big the effects are in your statistical results — something p-values can’t do.
But they’re only useful if you can choose the most appropriate one and if you can interpret it.
This can be hard in even simple statistical tests. But once you get into complicated models, it’s a whole new story. (more…)
Whenever we run an analysis of variance or run a regression one of the first things we do is look at the p-value of our predictor variables to determine whether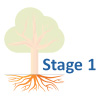
they are statistically significant. When the variable is statistically significant, did you ever stop and ask yourself how significant it is? (more…)
Effect size statistics are expected by many journal editors these days.
If you’re running an ANOVA, t-test, or linear regression model, it’s pretty straightforward which ones to report.
Things get trickier, though, once you venture into other types of models.
(more…)