Survival analysis isn’t just a single model.
It’s a whole set of tests, graphs, and models that are all used in slightly different data and study design situations. Choosing the most appropriate model can be challenging.
In this article I will describe the most common types of tests and models in survival analysis, how they differ, and some challenges to learning them.
(more…)
by Steve Simon, PhD
There are two features of survival models.
First is the process of measuring the time in a sample of people, animals, or machines until a specific event occurs. In fact, many people use the term “time to event analysis” or “event history analysis” instead of “survival analysis” to emphasize the broad range of areas where you can apply these techniques.
Second is the recognition that not everyone/everything in your sample will experience the event. Those not experiencing the event, either because the study ended before they had the event or because they were lost to follow-up, are classified as censored observations.
(more…)
When you have data measuring the time to an event, you can examine the relationship between various predictor variables and the time to the event using a Cox proportional hazards model.
In this webinar, you will see what a hazard function is and describe the interpretations of increasing, decreasing, and constant hazard. Then you will examine the log rank test, a simple test closely tied to the Kaplan-Meier curve, and the Cox proportional hazards model.
Note: This training is an exclusive benefit to members of the Statistically Speaking Membership Program and part of the Stat’s Amore Trainings Series. Each Stat’s Amore Training is approximately 90 minutes long.
(more…)
What is the relationship between predictors and whether and when an event will occur?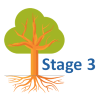
This is what event history (a.k.a., survival) analysis tests.
There are many flavors of Event History Analysis, though, depending on how time is measured, whether events can repeat, etc.
In this webinar, we discussed many of the issues involved in measuring time, including censoring, and introduce one specific type of event history model: the logistic model for discrete time events.
Note: This training is an exclusive benefit to members of the Statistically Speaking Membership Program and part of the Stat’s Amore Trainings Series. Each Stat’s Amore Training is approximately 90 minutes long.
(more…)
The first real data set I ever analyzed was from my senior honors thesis as an undergraduate psychology major. I had taken both intro stats and an ANOVA class, and I applied all my new skills with gusto, analyzing every which way.
It wasn’t too many years into graduate school that I realized that these data analyses were a bit haphazard and not at all well thought out. 20 years of data analysis experience later and I realized that’s just a symptom of being an inexperienced data analyst.
But even experienced data analysts can get off track, especially with large data sets with many variables. It’s just so easy to try one thing, then another, and pretty soon you’ve spent weeks getting nowhere.
(more…)
Censored data are inherent in any analysis, like Event History or Survival Analysis, in which the outcome measures the Time to Event TTE. Censoring occurs when the event doesn’t occur for an observed individual during the time we observe them.
Despite the name, the event of “survival” could be any categorical event that you would like to describe the mean or median TTE. To take the censoring into account, though, you need to make sure your data are set up correctly.
Here is a simple example, for a data set that measures days after surgery until an (more…)