Oops—you ran the analysis you planned to run on your data, carefully chosen to answer your research question, but your residuals aren’t normally distributed.
Maybe you’ve tried transforming the outcome variable, or playing around with the independent variables, but still no dice. That’s ok, because you can always turn to a non-parametric analysis, right?
Well, sometimes.
(more…)
Mixed models are hard.
They’re abstract, they’re a little weird, and there is not a common vocabulary or notation for them.
But they’re also extremely important to understand because many data sets require their use.
Repeated measures ANOVA has too many limitations. It just doesn’t cut it any more.
One of the most difficult parts of fitting mixed models is figuring out which random effects to include in a model. And that’s hard to do if you don’t really understand what a random effect is or how it differs from a fixed effect. (more…)
At The Analysis Factor, we are on a mission to help researchers improve their statistical skills so they can do amazing research.
We all tend to think of “Statistical Analysis” as one big skill, but it’s not.
Over the years of training, coaching, and mentoring data analysts at all stages, I’ve realized there are four fundamental stages of statistical skill:
Stage 1: The Fundamentals
Stage 2: Linear Models
Stage 3: Extensions of Linear Models
Stage 4: Advanced Models
There is also a stage beyond these where the mathematical statisticians dwell. But that stage is required for such a tiny fraction of data analysis projects, we’re going to ignore that one for now.
If you try to master the skill of “statistical analysis” as a whole, it’s going to be overwhelming.
And honestly, you’ll never finish. It’s too big of a field.
But if you can work through these stages, you’ll find you can learn and do just about any statistical analysis you need to. (more…)
Every statistical model and hypothesis test has assumptions.
And yes, if you’re going to use a statistical test, you need to check whether those assumptions are reasonable to whatever extent you can.
Some assumptions are easier to check than others. Some are so obviously reasonable that you don’t need to do much to check them most of the time. And some have no good way of being checked directly, so you have to use situational clues.
(more…)
When I was in graduate school, stat professors would say “ANOVA is just a special case of linear regression.” But they never explained why.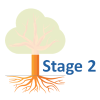
And I couldn’t figure it out.
The model notation is different.
The output looks different.
The vocabulary is different.
The focus of what we’re testing is completely different. How can they be the same model?
(more…)