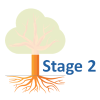
In your statistics class, your professor made a big deal about unequal sample sizes in one-way Analysis of Variance (ANOVA) for two reasons.
1. Because she was making you calculate everything by hand. Sums of squares require a different formula* if sample sizes are unequal, but statistical software will automatically use the right formula. So we’re not too concerned. We’re definitely using software.
2. Nice properties in ANOVA such as the Grand Mean being the intercept in an effect-coded regression model don’t hold when data are unbalanced. Instead of the grand mean, you need to use a weighted mean. That’s not a big deal if you’re aware of it. (more…)
If your graduate statistical training was anything like mine, you learned ANOVA in one class and Linear Regression in another. My professors would often say things like “ANOVA is just a special case of Regression,” but give vague answers when pressed.
It was not until I started consulting that I realized how closely related ANOVA and regression are. They’re not only related, they’re the same thing. Not a quarter and a nickel–different sides of the same coin.
So here is a very simple example that shows why. When someone showed me this, a light bulb went on, even though I already knew both ANOVA and multiple linear (more…)
Back when I was doing psychology research, I knew ANOVA pretty well. I’d taken a number of courses on it and could run it backward and forward. I kept hearing about ANCOVA, but in every ANOVA class that was the last topic on the syllabus, and we always ran out of time.
The other thing that drove me crazy was those stats professors kept saying “ANOVA is just a special case of Regression.” I could not for the life of me figure out why or how.
It was only when I switched over to statistics that I finally took a regression class and figured out what ANOVA was all about. And only when I started consulting, and seeing hundreds of different ANOVA and regression models, that I finally made the connection.
But if you don’t have the driving curiosity about ANOVA and regression, why should you, as a researcher in Psychology, Education, or Agriculture, who is trained in ANOVA, want to learn regression? There are 3 main reasons.
1. There a many, many continuous independent variables and covariates that need to be included in models. Without the tools to analyze them as continuous, you are left forcing them into ANOVA using an arbitrary technique like median splits. At best, you’re losing power. At worst, you’re not publishing your article because you’re missing real effects.
2. Having a solid understanding of the General Linear Model in its various forms equips you to really understand your variables and their relationships. It allows you to try a model different ways–not for data fishing, but for discovering the true nature of the relationships. Having the capacity to add an interaction term or a squared term allows you to listen to your data and makes you a better researcher.
3. The multiple linear regression model is the basis for many other statistical techniques–logistic regression, multilevel and mixed models, Poisson regression, Survival Analysis, and so on. Each of these is a step (or small leap) beyond multiple regression. If you’re still struggling with what it means to center variables or interpret interactions, learning one of these other techniques becomes arduous, if not painful.
Having guided thousands of researchers through their statistical analysis over the past 10 years, I am convinced that having a strong, intuitive understanding of the general linear model in its variety of forms is the key to being an effective and confident statistical analyst. You are then free to learn and explore other methodologies as needed.