The classic way to compare means in analysis of variance is examining pairwise differences in means after an F test.. It’s great for many problems, but sometimes your research question isn’t about pairwise differences.
Pairwise differences are not ideal if your research question is if, like the Sesame Street song, one of these groups is not like the others. Perhaps, you are trying to assure that groups conform to a common standard. Analysis of Means (ANOM) helps in all these settings. (more…)
Often a model is not a simple process from a treatment or intervention to the outcome. In essence, the value of X does not always directly predict the value of Y.
Mediators can affect the relationship between X and Y. Moderators can affect the scale and magnitude of that relationship. And sometimes the mediators and moderators affect each other.
There are many types and examples of ordinal variables: percentiles, ranks, likert scale items, to name a few.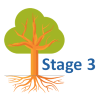
These are especially hard to know how to analyze–some people treat them as numerical, others emphatically say not to. Everyone agrees nonparametric tests work, but these are limited to testing only simple hypotheses and designs. So what do you do if you want to test something more elaborate?
In this webinar we’re going to lay out all the options and when each is (more…)
So you can’t randomize people into THAT condition? Now what?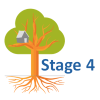
Let’s say you’re investigating the impact of smoking on social outcomes like depression, poverty, or quality of life. Your IRB, with good reason, won’t allow random assignment of smoking status to your participants.
But how can you begin to overcome the self selected nature of smoking among the study participants? What if self-selection is driving differences in outcomes? Well, one way is to use propensity score matching and analysis as a framework for your investigation.
The propensity score is the probability of group assignment conditional on observed baseline characteristics. In this way, the propensity score is a balancing score: conditional on the propensity score, the distribution of observed baseline covariates will be similar between treated and untreated subjects.
In this webinar, we’ll describe broadly what this method is and discuss different matching methods that can be used to create balanced samples of “treated” and “non-treated” participants. Finally, we’ll discuss some specific software resources that can be found to perform these analyses.
Note: This training is an exclusive benefit to members of the Statistically Speaking Membership Program and part of the Stat’s Amore Trainings Series. Each Stat’s Amore Training is approximately 90 minutes long.
(more…)
P-values are the fundamental tools used in most inferential data analyses
(more…)