online workshops
Structural Equation Modeling
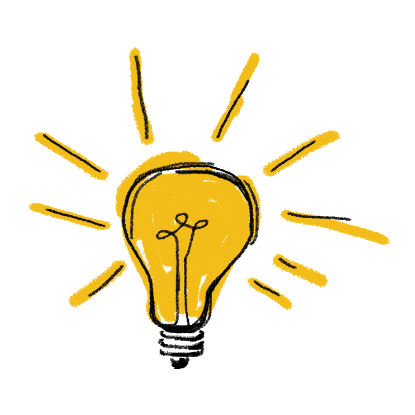
Even though it’s a relative newcomer to the field of statistics, Structural Equation Modeling (SEM) has gained rapid popularity as a powerful statistical tool. This flexible analysis framework allows researchers to examine the set of relationships between one or more independent variables and one or more dependent variables, and, to analyze the structural relationship between measured variables and latent constructs. learn more
statistically speaking member trainings
Introduction to Structural Equation Modeling
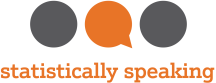
Structural Equation Modeling (SEM) is a popular method to test hypothetical relationships between constructs in the social sciences. These constructs may be unobserved (a.k.a., “latent”) or observed (a.k.a., “manifest”). learn more
Model Fit Statistics in Structural Equation Modeling
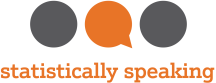
Structural Equation Modeling (SEM) increasingly is a ‘must’ for researchers in the social sciences and business analytics. However, the issue of how consistent the theoretical model is with the data, known as model fit, is by no means agreed upon: There is an abundance of fit indices available – and wide disparity in agreement on which indices to report and what the cut-offs for various indices actually are. learn more
Reporting Structural Equation Modeling Results
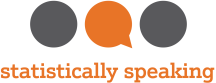
The last, and sometimes hardest, step for running any statistical model is writing up results. As with most other steps, this one is a bit more complicated for structural equation models than it is for simpler models like linear regression. learn more
A Guide to Latent Variable Models
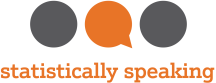
An extremely useful area of statistics is a set of models that use latent variables: variables whole values we can’t measure directly, but instead have to infer from others. These latent variables can be unknown groups, unknown numerical values, or unknown patterns in trajectories. learn more
the craft of statistical analysis free webinars
Three Overlooked Strengths of Structural Equation Modeling
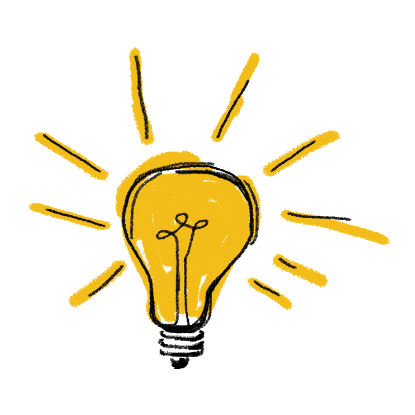
Confirmatory factor analysis (CFA) and path models make up two core building blocks of SEM. When you understand each one and how they can work together, you can analyze data in ways not possible using regression. learn more
articles at the analysis factor
First Steps in Structural Equation Modeling: Confirmatory Factor Analysis
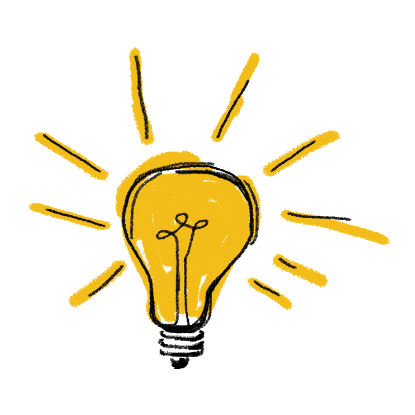
Confirmatory factor analysis (CFA) is the fundamental first step in running most types of SEM models. You want to do this first to verify the measurement quality of any and all latent constructs you’re using in your structural equation model. The term “regression” is an umbrella for numerous statistical methods. learn more
One of the Many Advantages to Running Confirmatory Factor Analysis with a Structural Equation Model
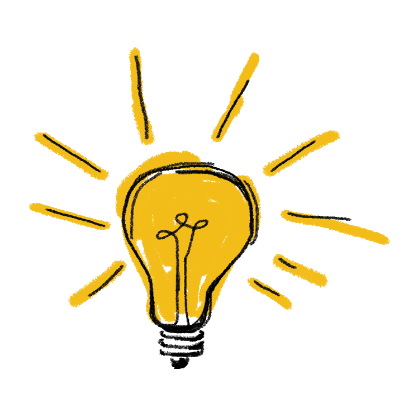
Based on questions I’ve been asked by clients, most analysts prefer using the factor analysis procedures in their general statistical software to run a confirmatory factor analysis. While this can work in some situations, you’re losing out on some key information you’d get from a structural equation model. learn more
The Four Models You Meet in Structural Equation Modeling
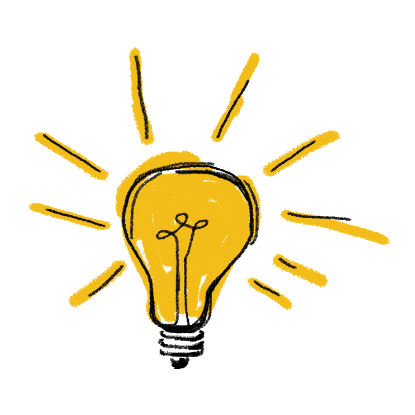
Previously, we showed how a multiple regression model could be conceptualized using Structural Equation Model path diagrams. That’s the simplest SEM you can create, but its real power lies in expanding on that regression model. Here I discuss four ways to do that. learn more
Five Things You Need to Know Before Learning Structural Equation Modeling
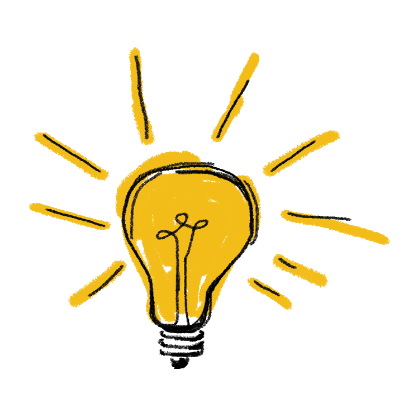
If you already know the principles of general linear modeling (GLM) you are on the right path to understand Structural Equation Modeling (SEM). SEM offers the flexibility of adding paths between predictors in a way that would take you several GLM models and still leave you with unanswered questions. learn more
Structural Equation Modeling: What is a Latent Variable?
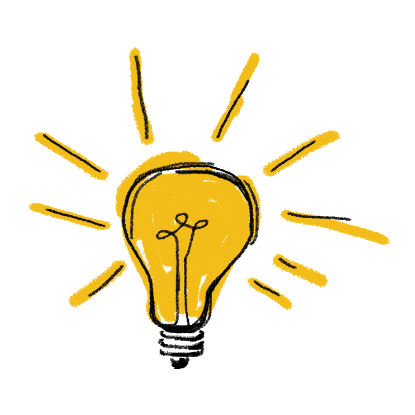
What is a latent variable? My favourite image to explain the relationship between latent and observed variables comes from the “Myth of the Cave” from Plato’s The Republic. In this myth a group of people are constrained to face a wall. The only things they see are shadows of objects that pass in front of a fire behind them. learn more
Why Adding Values on a Scale Can Lead to Measurement Error
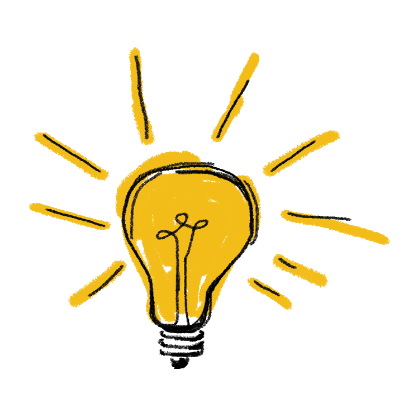
Whenever you use a multi-item scale to measure a construct, a key step is to create a score for each subject in the data set. This score is an estimate of the value of the latent construct (factor) the scale is measuring for each subject. In fact, calculating this score is the final step of running a Confirmatory Factor Analysis. learn more
Correlated Errors in Confirmatory Factor Analysis
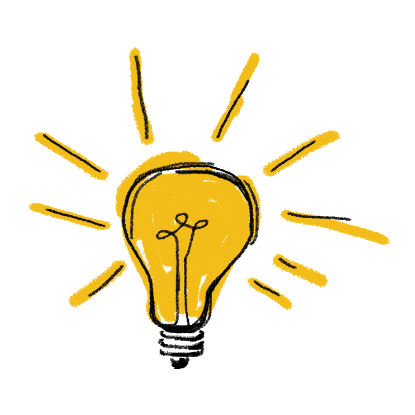
Latent constructs, such as liberalism or conservatism, are theoretical and cannot be measured directly. But we can represent the latent construct by combining a set of questions on a scale, called indicators. We do this via factor analysis. learn more
Confirmatory Factor Analysis: How To Measure Something We Cannot Observe or Measure Directly
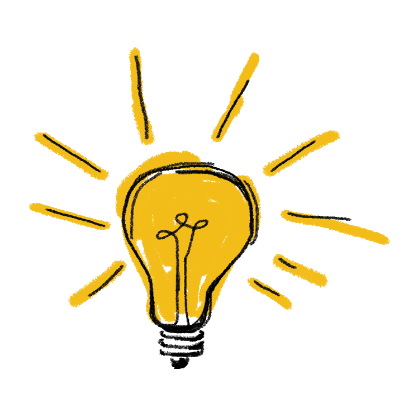
Many times in science we are intrigued to measure an underlying characteristic that cannot be observed or measured directly. This measure is hypothesized to exist to explain variables, such as behavior, that can be observed. The measurable variables are called manifest variables. The unmeasurable are called latent variables. learn more
Why Do I Need to Have Knowledge of Multiple Regression to Understand SEM?
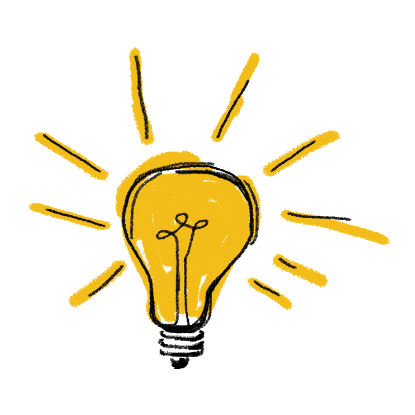
General Linear Model (GLM) is a tool used to understand and analyse linear relationships among variables. It is an umbrella term for many techniques that are taught in most statistics courses: ANOVA, multiple regression, etc. In its simplest form it describes the relationship between two variables, “y” (dependent variable, outcome, and so on) and “x” (independent variable, predictor, etc). learn more