online workshops
Principal Component Analysis and Exploratory Factor Analysis
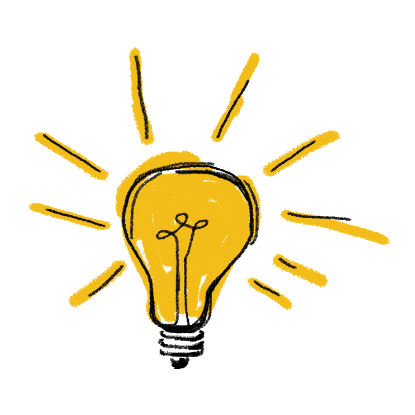
Are you ready to tackle multivariate statistics, but find yourself overwhelmed? When you’re working with many correlated variables, they get too unwieldy to use individually. But all of them are important to measure. learn more
the craft of statistical analysis free webinars
Principal Component Analysis
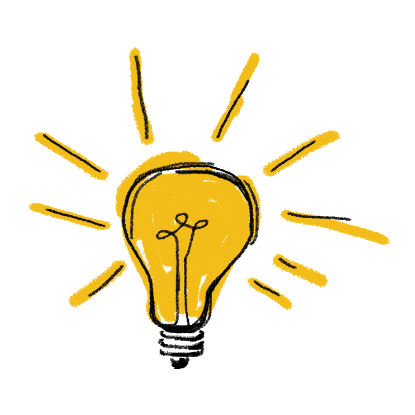
Principal Component Analysis is a variable reduction procedure – it allows you summarize the common variation in many variables into just a few. It’s similar to Factor Analysis, but has different underlying assumptions. learn more
statistically speaking member trainings
Confirmatory Factor Analysis
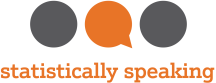
There are two main types of factor analysis: exploratory and confirmatory. Exploratory factor analysis (EFA) is data driven, such that the collected data determines the resulting factors. Confirmatory factor analysis (CFA) is used to test factors that have been developed a priori. Think of CFA as a process for testing what you already think you know. learn more
Exploratory Factor Analysis
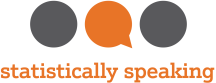
Many variables we want to measure just can’t be directly measured with a single variable. Instead you have to combine a set of variables into a single index. But how do you determine which variables to combine and how best to combine them? learn more
articles at the analysis factor
introduction to factor analysis series
Factor Analysis: A Short Introduction
Part 1
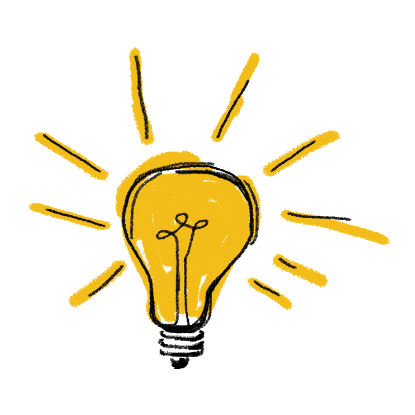
Factor analysis is a useful tool for investigating variable relationships for complex concepts such as socioeconomic status, dietary patterns, or psychological scales. It allows researchers to investigate concepts they cannot measure directly. It does this by using a large number of variables to esimate a few interpretable underlying factors. learn more
Factor Analysis: A Short Introduction
Part 2 – Rotations
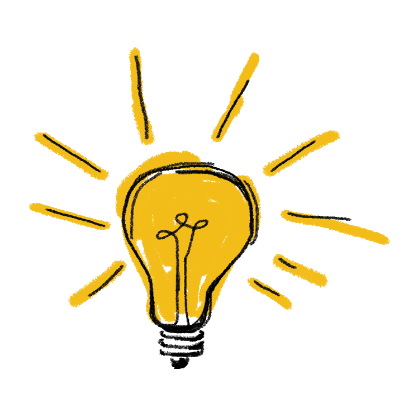
An important feature of factor analysis is that the axes of the factors can be rotated within the multidimensional variable space. What does that mean? Here is, in simple terms, what a factor analysis program does while determining the best fit between the variables and the latent factors. learn more
Factor Analysis: A Short Introduction
Part 3 – The Difference Between Confirmatory and Exploratory Factor Analysis
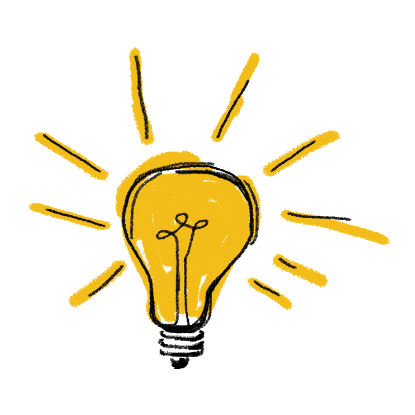
An important question that the consultants at The Analysis Factor are frequently asked is, “What is the difference between a confirmatory and an exploratory factor analysis?” A confirmatory factor analysis assumes that you enter the factor analysis with a firm idea about the number of factors you will encounter, and about which variables will most likely load onto each factor. learn more
Factor Analysis: A Short Introduction
Part 4 – How Many Factors Should I Find?
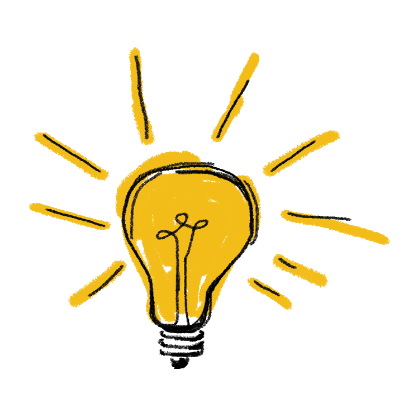
One of the hardest things to determine when conducting a factor analysis is how many factors to settle on. Statistical programs provide a number of criteria to help with the selection. Programs usually have a default cut-off for the number of generated factors, such as all factors with an eigenvalue of ≥1. learn more
Factor Analysis: A Short Introduction
Part 5 – Dropping Unimportant Variables From Your Analysis
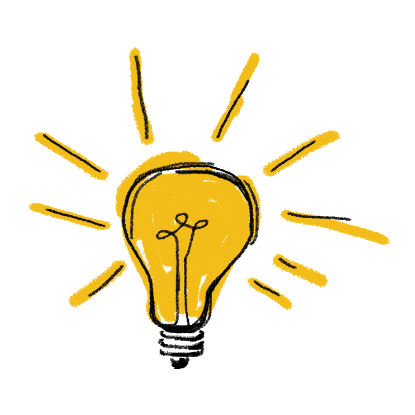
Once you run a factor analysis and think you have some usable results, it’s time to eliminate variables that are not “strong” enough. They are usually the ones with low factor loadings, although additional criteria should be considered before taking out a variable. learn more
Factor Analysis: A Short Introduction
Part 6 – Common Problems
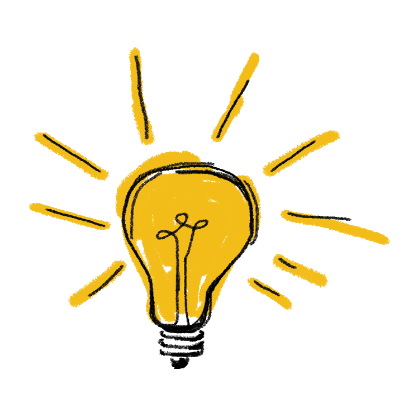
In the previous blogs I wrote about the basics of running a factor analysis. Real-life factor analysis can become complicated. Here are some of the more common problems researchers encounter and some possible solutions. learn more
specific issues in pca and fa
In Factor Analysis, How Do We Decide Whether to Have Rotated or Unrotated Factors?
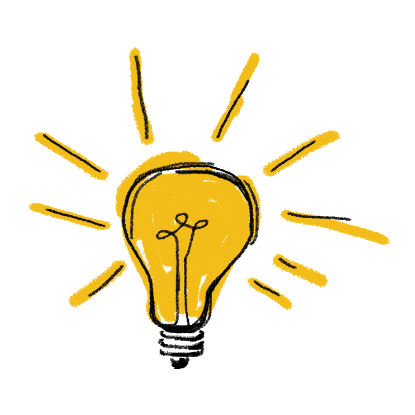
I recently gave a free webinar on Principal Component Analysis. We had almost 300 researchers attend and didn’t get through all the questions. This is part of a series of answers to those questions. learn more
The Fundamental Difference Between Principal Component Analysis and Factor Analysis
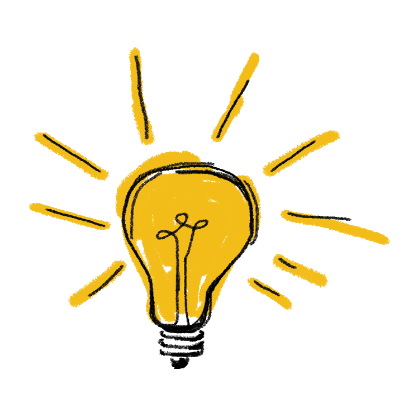
One of the many confusing issues in statistics is the confusion between Principal Component Analysis (PCA) and Factor Analysis (FA). They are very similar in many ways, so it’s not hard to see why they’re so often confused. They appear to be different varieties of the same analysis rather than two different methods. learn more
How To Calculate an Index Score from a Factor Analysis
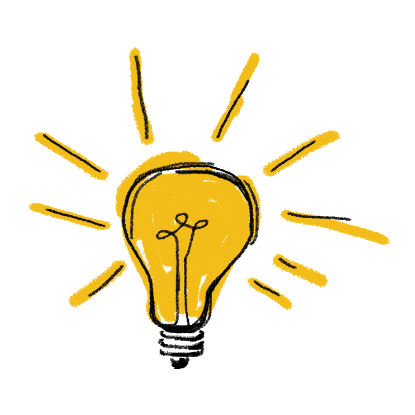
One common reason for running Principal Component Analysis (PCA) or Factor Analysis (FA) is variable reduction. In other words, you may start with a 10-item scale meant to measure something like Anxiety, which is difficult to accurately measure with a single question. learn more
Can You Use Principal Component Analysis with a Training Set Test Set Model?
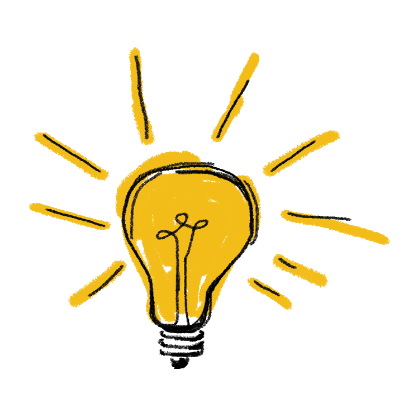
I recently gave a free webinar on Principal Component Analysis. We had almost 300 researchers attend and didn’t get through all the questions. This is part of a series of answers to those questions. learn more
In Principal Component Analysis, Can Loadings Be Negative?
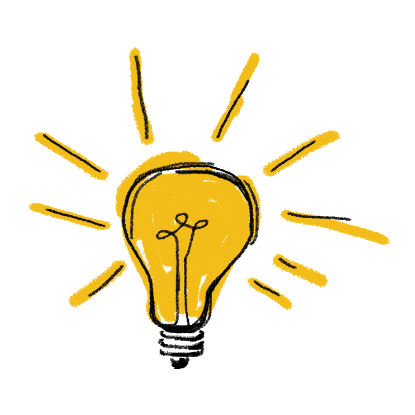
Here’s a question I get pretty often: In Principal Component Analysis, can loadings be negative and positive? Answer: Yes. Recall that in PCA, we are creating one index variable (or a few) from a set of variables. You can think of this index variable as a weighted average of the original variables. learn more
Principal Component Analysis for Ordinal Scale Items
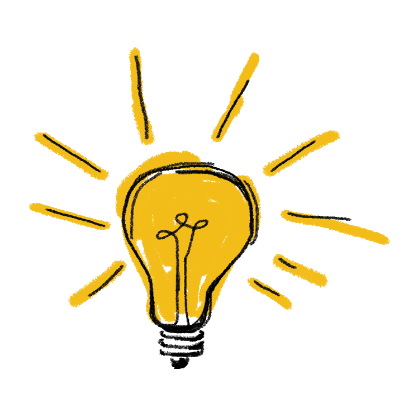
Principal Component Analysis is really, really useful. You use it to create a single index variable from a set of correlated variables. In fact, the very first step in Principal Component Analysis is to create a correlation matrix (a.k.a., a table of bivariate correlations). The rest of the analysis is based on this correlation matrix. learn more
Three Tips for Principal Component Analysis
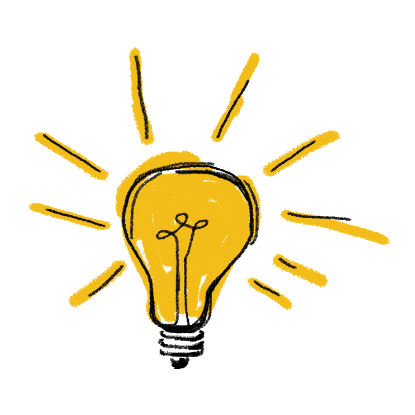
Principal Component Analysis (PCA) is a handy statistical tool to always have available in your data analysis tool belt. It’s a data reduction technique, which means it’s a way of capturing the variance in many variables in a smaller, easier-to-work-with set of variables. learn more
Can We Use PCA for Reducing Both Predictors and Response Variables?
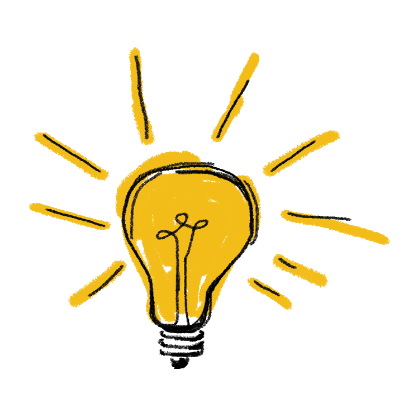
I recently gave a free webinar on Principal Component Analysis. We had almost 300 researchers attend and didn’t get through all the questions. This is part of a series of answers to those questions. learn more
How to Reduce the Number of Variables to Analyze
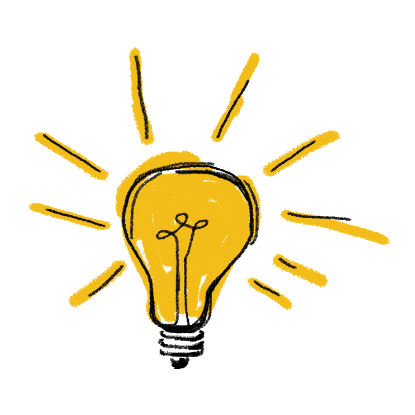
Many data sets contain well over a thousand variables. Such complexity, the speed of contemporary desktop computers, and the ease of use of statistical analysis packages can encourage ill-directed analysis. It is easy to generate a vast array of poor “results” by throwing everything into your software and waiting to see what turns up. learn more
Correlated Errors in Confirmatory Factor Analysis
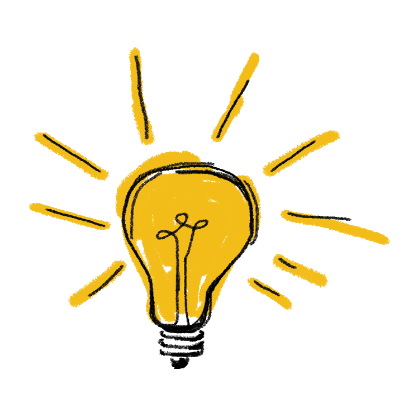
Latent constructs, such as liberalism or conservatism, are theoretical and cannot be measured directly. But we can represent the latent construct by combining a set of questions on a scale, called indicators. We do this via factor analysis. learn more
Life After Exploratory Factor Analysis: Estimating Internal Consistency
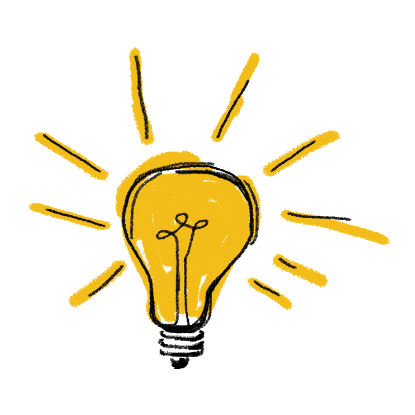
After you are done with the odyssey of exploratory factor analysis (aka a reliable and valid instrument), you may find yourself at the beginning of a journey rather than the ending. The process of performing exploratory factor analysis usually seeks to answer whether a given set of items form a coherent factor (or often several factors). learn more
Confirmatory Factor Analysis: How To Measure Something We Cannot Observe or Measure Directly
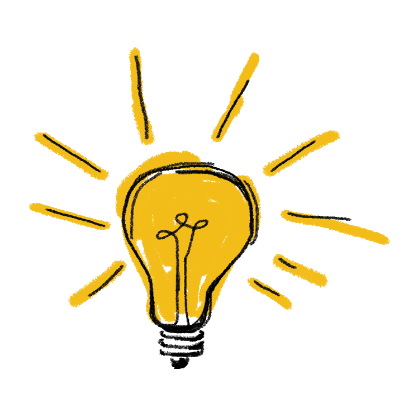
Many times in science we are intrigued to measure an underlying characteristic that cannot be observed or measured directly. This measure is hypothesized to exist to explain variables, such as behavior, that can be observed. The measurable variables are called manifest variables. The unmeasurable are called latent variables. learn more
Three Myths and Truths About Model Fit in Confirmatory Factor Analysis
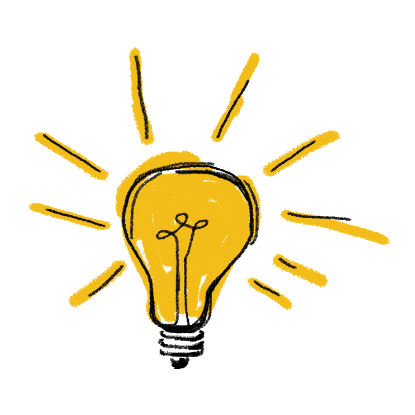
We mentioned before that we use Confirmatory Factor Analysis to evaluate whether the relationships among the variables are adequately represented by the hypothesized factor structure. The factor structure (relationships between factors and variables) can be based on theoretical justification or previous findings. learn more
Four Common Misconceptions in Exploratory Factor Analysis
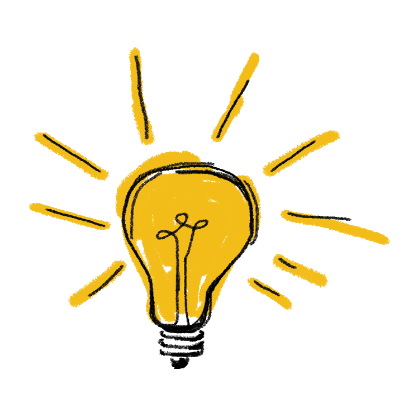
Here, I would like to briefly describe four misconceptions that I feel are commonly perceived by novice researchers in Exploratory Factor Analysis. learn more