Many who work with statistics are already functionally familiar with the normal distribution, and maybe even the binomial distribution.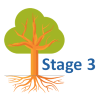
These common distributions are helpful in many applications, but what happens when they just don’t work?
This webinar will cover a number of statistical distributions, including the:
- Poisson and negative binomial distributions (especially useful for count data)
- Multinomial distribution (for responses with more than two categories)
- Beta distribution (for continuous percentages)
- Gamma distribution (for right-skewed continuous data)
- Bernoulli and binomial distributions (for probabilities and proportions)
- And more!
We’ll also explore the relationships among statistical distributions, including those you may already use, like the normal, t, chi-squared, and F distributions.
Note: This training is an exclusive benefit to members of the Statistically Speaking Membership Program and part of the Stat’s Amore Trainings Series. Each Stat’s Amore Training is approximately 90 minutes long.
(more…)
One of the most common—and one of the trickiest—challenges in data analysis is deciding how to include multiple predictors in a model, especially when they’re related to each other.
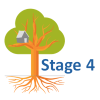
Let’s say you are interested in studying the relationship between work spillover into personal time as a predictor of job burnout.
You have 5 categorical yes/no variables that indicate whether a particular symptom of work spillover is present (see below).
While you could use each individual variable, you’re not really interested if one in particular is related to the outcome. Perhaps it’s not really each symptom that’s important, but the idea that spillover is happening.
(more…)
There are two main types of factor analysis: exploratory and confirmatory. Exploratory factor analysis (EFA) is data driven, such that the collected data determines the resulting factors.
Confirmatory factor analysis (CFA) is used to test factors that have been developed a priori.
Think of CFA as a process for testing what you already think you know.
CFA is an integral part of structural equation modeling (SEM) and path analysis. The hypothesized factors should always be validated with CFA in a measurement model prior to incorporating them into a path or structural model. Because… garbage in, garbage out.
CFA is also a useful tool in checking the reliability of a measurement tool with a new population of subjects, or to further refine an instrument which is already in use.
Elaine will provide an overview of CFA. She will also (more…)
The LASSO model (Least Absolute Shrinkage and Selection Operator) is a recent development that allows you to find a good fitting model in the regression context. It avoids many of the problems
of overfitting that plague other model-building approaches.
In this Statistically Speaking Training, guest instructor Steve Simon, PhD, explains what overfitting is — and why it’s a problem.
Then he illustrates the geometry of the LASSO model in comparison to other regression approaches, ridge regression and stepwise variable selection.
Finally, he shows you how LASSO regression works with a real data set.
Note: This training is an exclusive benefit to members of the Statistically Speaking Membership Program and part of the Stat’s Amore Trainings Series. Each Stat’s Amore Training is approximately 90 minutes long.
(more…)
Statistically speaking, when we see a continuous outcome variable we often worry about outliers and how these extreme observations can impact our model.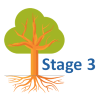
But have you ever had an outcome variable with no outliers because there was a boundary value at which accurate measurements couldn’t be or weren’t recorded?
Examples include:
- Income data where all values above $100,000 are recorded as $100k or greater
- Soil toxicity ratings where the device cannot measure values below 1 ppm
- Number of arrests where there are no zeros because the data set came from police records where all participants had at least one arrest
These are all examples of data that are truncated or censored. Failing to incorporate the truncation or censoring will result in biased results.
This webinar will discuss what truncated and censored data are and how to identify them.
There are several different models that are used with this type of data. We will go over each model and discuss which type of data is appropriate for each model.
We will then compare the results of models that account for truncated or censored data to those that do not. From this you will see what possible impact the wrong model choice has on the results.
Note: This training is an exclusive benefit to members of the Statistically Speaking Membership Program and part of the Stat’s Amore Trainings Series. Each Stat’s Amore Training is approximately 90 minutes long.
(more…)
Survival data models provide interpretation of data representing the time until an event occurs. In many situations, the event is death, but it can also represent the time to other bad events such as
cancer relapse or failure of a medical device. It can also be used to denote time to positive events such as pregnancy. Often patients are lost to follow-up prior to death, but you can still use the information about them while they were in your study to better estimate the survival probability over time.
This is done using the Kaplan-Meier curve, an approach developed by (more…)