While parametric regression models like linear and logistic regression are still the mainstay of statistical modeling, they are not the only, nor always the best, approach to predicting outcome
variables.
Classification and Regression Trees (CART) are a nonparametric approach to using values of predictors to find good predictions of values of a response variable.
On each step, the values of a predictor variable are optimally split such that they predict the values of the response variable. The set of splits across multiple predictors leads to a tree.
CART models work for either categorical or numerical response variables and predictor variables, and they are especially good at revealing complex interactions among predictors. So they work well as either an exploratory technique before or a predictive model instead of logistic or linear regression.
In this webinar, we’ll explore CART modelling and discuss what it is, which options work when, and how to interpret the output.
Note: This training is an exclusive benefit to members of the Statistically Speaking Membership Program and part of the Stat’s Amore Trainings Series. Each Stat’s Amore Training is approximately 90 minutes long.
About the Instructor
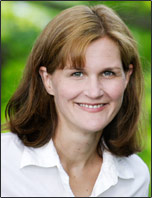
Karen Grace-Martin helps statistics practitioners gain an intuitive understanding of how statistics is applied to real data in research studies.
She has guided and trained researchers through their statistical analysis for over 15 years as a statistical consultant at Cornell University and through The Analysis Factor. She has master’s degrees in both applied statistics and social psychology and is an expert in SPSS and SAS.
Not a Member Yet?
It’s never too early to set yourself up for successful analysis with support and training from expert statisticians.
Just head over and sign up for Statistically Speaking.
You'll get access to this training webinar, 130+ other stats trainings, a pathway to work through the trainings that you need — plus the expert guidance you need to build statistical skill with live Q&A sessions and an ask-a-mentor forum.
Cluster analysis classifies individuals into two or more unknown groups based on a set of numerical variables.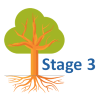
It is related to, but distinct from, a few other multivariate techniques including discriminant Function Analysis, (more…)
What is the relationship between predictors and whether and when an event will occur?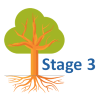
This is what event history (a.k.a., survival) analysis tests.
There are many flavors of Event History Analysis, though, depending on how time is measured, whether events can repeat, etc.
In this webinar, we discussed many of the issues involved in measuring time, including censoring, and introduce one specific type of event history model: the logistic model for discrete time events.
Note: This training is an exclusive benefit to members of the Statistically Speaking Membership Program and part of the Stat’s Amore Trainings Series. Each Stat’s Amore Training is approximately 90 minutes long.
(more…)
Complex Surveys use a sampling technique other than a simple random sample. Terms you may have heard in this area include cluster sampling, stratified sampling, oversampling, two-stage
sampling, and primary sampling unit.
Complex Samples require statistical methods that take the exact sampling design into account to ensure accurate results.
In this webinar, guest instructor Dr. Trent Buskirk will give you an overview of the common sampling techniques and their effects on data analysis.
Note: This training is an exclusive benefit to members of the Statistically Speaking Membership Program and part of the Stat’s Amore Trainings Series. Each Stat’s Amore Training is approximately 90 minutes long.
About the Instructor
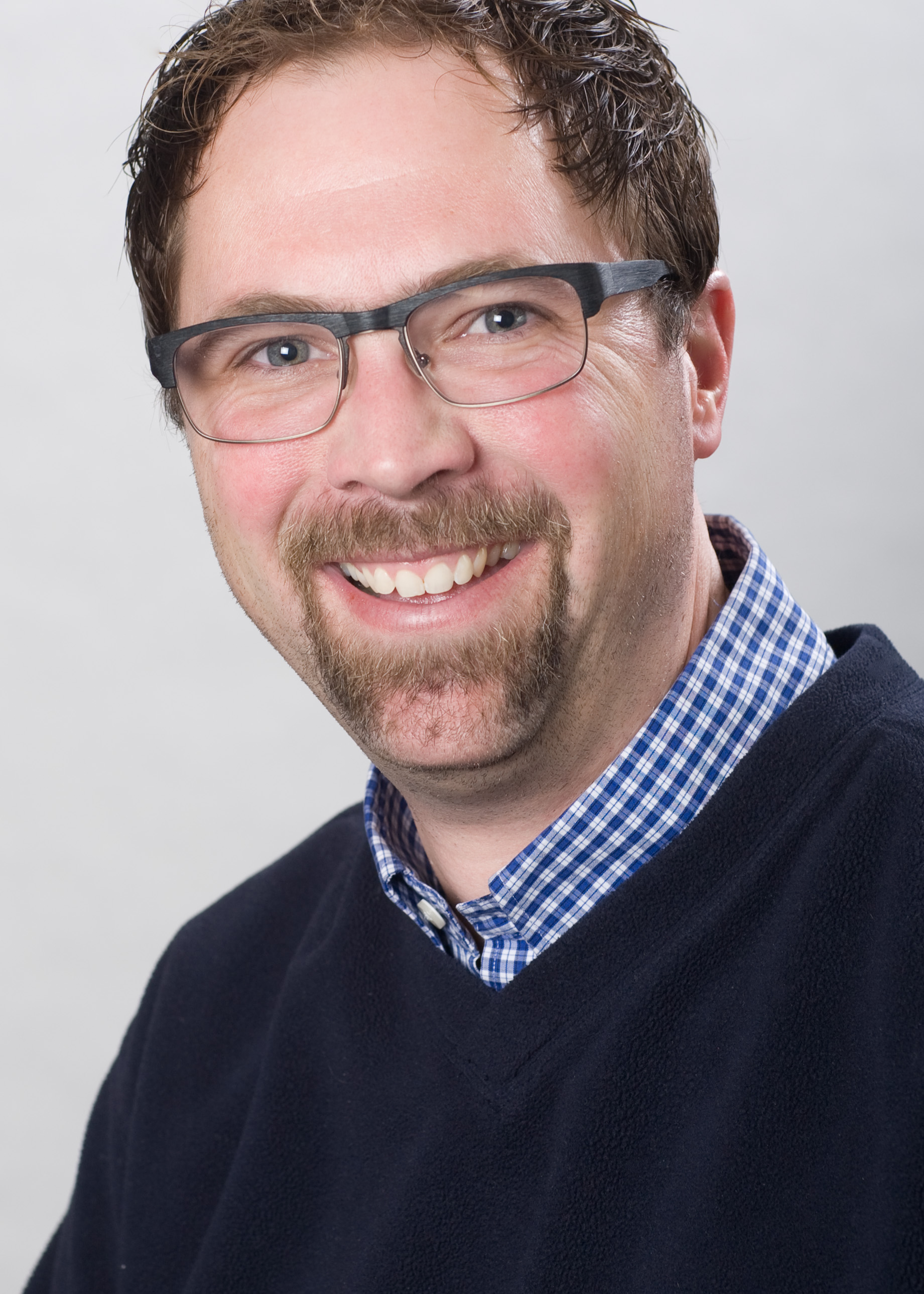
Trent D. Buskirk, Ph.D. is the Vice President of Statistics and Methodology, Marketing Systems Group.
Dr. Buskirk has more than 15 years of professional and academic experience in the fields of survey research, statistics, as well as SPSS, SAS, and R.
Dr. Buskirk has taught for more than a decade at the University of Nebraska and Saint Louis University where he was an Associate Professor of Biostatistics in the School of Public Health.
Not a Member Yet?
It’s never too early to set yourself up for successful analysis with support and training from expert statisticians.
Just head over and sign up for Statistically Speaking.
You'll get access to this training webinar, 130+ other stats trainings, a pathway to work through the trainings that you need — plus the expert guidance you need to build statistical skill with live Q&A sessions and an ask-a-mentor forum.
Mediation indicates that a mediator M is the causal link in the effect between an independent variable X and a dependent variable Y.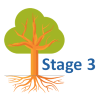
How to test mediation has become a very hot topic of statistical research in the past 10 years, and there are many new advances.
In this webinar, we’ll discuss a number of issues, new and old, in mediation, including the Baron & Kenny 4-step approach, the difference between mediation and moderation, Sobel and bootstrap tests for indirect effects. (And of course, what all these terms mean.)
Note: This training is an exclusive benefit to members of the Statistically Speaking Membership Program and part of the Stat’s Amore Trainings Series. Each Stat’s Amore Training is approximately 90 minutes long.
About the Instructor
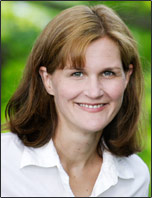
Karen Grace-Martin helps statistics practitioners gain an intuitive understanding of how statistics is applied to real data in research studies.
She has guided and trained researchers through their statistical analysis for over 15 years as a statistical consultant at Cornell University and through The Analysis Factor. She has master’s degrees in both applied statistics and social psychology and is an expert in SPSS and SAS.
Not a Member Yet?
It’s never too early to set yourself up for successful analysis with support and training from expert statisticians.
Just head over and sign up for Statistically Speaking.
You'll get access to this training webinar, 130+ other stats trainings, a pathway to work through the trainings that you need — plus the expert guidance you need to build statistical skill with live Q&A sessions and an ask-a-mentor forum.
Item Response Theory (IRT) refers to a family of statistical models for evaluating the design and scoring of psychometric tests, assessments and surveys. It is used on assessments in psychology,
psychometrics, education, health studies, marketing, economics and social sciences — assessments that involve categorical items (e.g., Likert items).
In this webinar, you will learn about:
- The key ideas and techniques of IRT, with examples from educational assessment
- The Rasch Model and the Graded Response Model — two of the most commonly-used IRT models
- A range of analytic techniques that should be used in conjunction with IRT
This webinar will introduce you to the basic ideas and applications of IRT, and show how you can acquire the skills necessary to conduct IRT analysis at the professional level.
Note: This training is an exclusive benefit to members of the Statistically Speaking Membership Program and part of the Stat’s Amore Trainings Series. Each Stat’s Amore Training is approximately 90 minutes long.
About the Instructor
David Lillis is an applied statistician in Wellington, New Zealand.
His company, Sigma Statistics and Research Limited, provides online instruction, face-to-face workshops on R, and coding services in R.
David holds a doctorate in applied statistics and is a frequent contributor to The Analysis Factor, including our blog series R is Not So Hard.
Not a Member Yet?
It’s never too early to set yourself up for successful analysis with support and training from expert statisticians.
Just head over and sign up for Statistically Speaking.
You'll get access to this training webinar, 130+ other stats trainings, a pathway to work through the trainings that you need — plus the expert guidance you need to build statistical skill with live Q&A sessions and an ask-a-mentor forum.