Regression models are just a subset of the General Linear Model, so you can use GLM procedures to run regressions. It is what I usually use.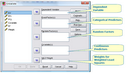
But in SPSS there are options available in the GLM and Regression procedures that aren’t available in the other. How do you decide when to use GLM and when to use Regression?
GLM has these options that Regression doesn’t: (more…)
A polynomial term–a quadratic (squared) or cubic (cubed) term turns a linear regression model into a curve. But because it is X that is squared or cubed, not the Beta coefficient, it still qualifies as a linear model. This makes it a nice, straightforward way to model curves without having to model complicated non-linear models.
But how do you know if you need one–when a linear model isn’t the best model? (more…)
In a Regression model, should you drop interaction terms if they’re not significant?
In an ANOVA, adding interaction terms still leaves the main effects as main effects. That is, as long as the data are balanced, the main effects and the interactions are independent. The main effect is still telling (more…)
A Linear Regression Model with an interaction between two predictors (X1 and X2) has the form: 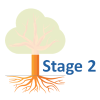
Y = B0 + B1X1 + B2X2 + B3X1*X2.
It doesn’t really matter if X1 and X2 are categorical or continuous, but let’s assume they are continuous for simplicity.
One important concept is that B1 and B2 are not main effects, the way they would be if (more…)
There are two reasons to center predictor variables in any type of regression analysis–linear, logistic, multilevel, etc.
1. To lessen the correlation between a multiplicative term (interaction or polynomial term) and its component variables (the ones that were multiplied).
2. To make interpretation of parameter estimates easier.
I was recently asked when is centering NOT a good idea? (more…)
I just discovered something in SPSS GLM that I never knew.
When you have an interaction in the model, the order you put terms into the Model statement affects which parameters SPSS gives you.
The default in SPSS is to automatically create interaction terms among all the categorical predictors. But if you want fewer than all those interactions, or if you want to put in an interaction involving a continuous variable, you need to choose Model–>Custom Model.
In the specific example of an interaction between a categorical and continuous variable, to interpret this interaction you need to output Regression Coefficients. Do this by choosing Options–>Regression Parameter Estimates.
If you put the main effects into the model first, followed by interactions, you will find the usual output–the regression coefficients (column B) for the continuous variable is the slope for the reference group. The coefficients for the interactions in the other categories tell you the difference between the slope for that category and the slope for the reference group. The coefficient for the reference group here in the interaction is 0.
What I was surprised to find is that if the interactions are put into the model first, you don’t get that.
Instead, the coefficients for the interaction of each category is the actual slope for that group, NOT the difference.
This is actually quite useful–it can save a bit of calculating and now you have a p-value for whether each slope is different from 0. However, it also means you have to be cautious and make sure you realize what each parameter estimate is actually estimating.