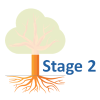
A normally distributed variable can have values without limits in both directions on the number line. While most variables have practical limitations, most of the time, this assumption of infinite tails is quite reasonable as there is no real boundary.
Air temperature is an example of a variable that can extend far from its mean in either direction.
But for other variables, there is a practical beginning or ending point. Age is left-bounded. It starts at zero.
The number of wins that a baseball team can have in a season is bounded on the upper end by the number of games played in a season.
The temperature of water as a liquid is bound on the low end at zero degrees Celsius and on the high end at 100 degrees Celsius.
There are two types of bounded data that have direct implications for how to work with them in analysis: censored and truncated data. Understanding the difference is a critical first step when working with these variables.
Understanding Censored and Truncated Data
Censored Data
Censored data have unknown values beyond a bound on either end of the number line or both. It can exist by design. When the data is observed and reported at the boundary, the researcher has made the decision to restrict the range of the scale.
An example of a lower censoring boundary is the recording of pollutants in our water. The researcher may not care about (or instruments may not be able to detect) the level of pollutants if it falls below a certain threshold (e.g., .005 parts per million). In this case, any pollutant level below .005 ppm is reported as “<.005 ppm.”
An upper censor could be placed on temperature in a science experiment. Once the temperature goes above x degrees the scientist doesn’t care. So s/he measures it as “>x”.
Data can be censored on both ends as well. Income could be reported as “<$20,000” if the actual is below $20,000 and reported as “ >$200,000” if above that level.
There are potential censored data not created by design. Test scores or college admission tests are examples of censored data not created by design, but by the actual bounds. A student cannot score above 100% correct no matter how much better they know the topic than other students. These are bounded by actual results.
Truncated Data
Truncation occurs when values beyond a boundary are either excluded when gathered or excluded when analyzed. For example, if someone conducting a survey asks you if you make more than $100,000, and you answer “yes” and the surveyor says “thanks but no thanks”, then you’ve been truncated.
Or if a number of arrests is measured from police records, then everyone with 0 arrests will, by definition, be excluded from the sample.
Excluding cases from a data set at a preset boundary has the same effect. Creating models on middle income values would involve truncating income above and below specific amounts.
So to summarize, data are censored when we have partial information about the value of a variable—we know it is beyond some boundary, but not how far above or below it.
In contrast, data are truncated when the data set does not include observations in the analysis that are beyond a boundary value. Having a value beyond the boundary eliminates that individual from being in the analysis.
In truncation, it’s not just the variable of interest that we don’t have full data on. It’s all the data from that case.
Jeff Meyer is a statistical consultant with The Analysis Factor, a stats mentor for Statistically Speaking membership, and a workshop instructor. Read more about Jeff here.
Go to the next article or see the full series on Easy-to-Confuse Statistical Concepts
Outliers are one of those realities of data analysis that no one can avoid.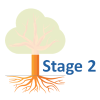
Those pesky extreme values cause biased parameter estimates, non-normality in otherwise beautifully normal variables, and inflated variances.
Everyone agrees that outliers cause trouble with parametric analyses. But not everyone agrees that they’re always a problem, or what to do about them even if they are.
Sometimes a nonparametric or robust alternative is available — and sometimes not.
There are a number of approaches in statistical analysis for dealing with outliers and the problems they create. It’s common for committee members or Reviewer #2 to have very strong opinions that there is one and only one good approach.
Two approaches that I’ve commonly seen are: 1) delete outliers from the sample, or 2) winsorize them (i.e., replace the outlier value with one that is less extreme).
The problem with both of these “solutions” is that they also cause problems — biased parameter estimates and underweighted or eliminated valid values. (more…)
There are not a lot of statistical methods designed just for ordinal variables.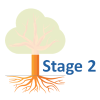
But that doesn’t mean that you’re stuck with few options. There are more than you’d think. (more…)
You put a lot of work into preparing and cleaning your data. Running the model is the moment of excitement.
You look at your tables and interpret the results. But first you remember that one or more variables had a few outliers. Did these outliers impact your results? (more…)
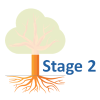
In a previous post , Using the Same Sample for Different Models in Stata, we examined how to use the same sample when comparing regression models. Using different samples in our models could lead to erroneous conclusions when interpreting results.
But excluding observations can also result in inaccurate results.
The coefficient for the variable “frequent religious attendance” was negative 58 in model 3 (more…)
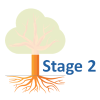
In my last article, Hierarchical Regression in Stata: An Easy Method to Compare Model Results, I presented the following table which examined the impact several predictors have on one’ mental health.
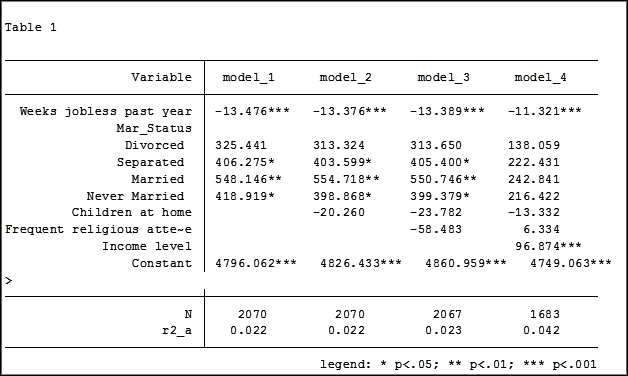
At the bottom of the table is the number of observations (N) contained within each sample.
The sample sizes are quite large. Does it really matter that they are different? The answer is absolutely yes.
Fortunately in Stata it is not a difficult process to use the same sample for all four models shown above.
Some background info:
As I have mentioned previously, Stata stores results in temp files. You don’t have to do anything to cause Stata to store these results, but if you’d like to use them, you need to know what they’re called.
To see what is stored after an estimation command, use the following code:
ereturn list
After a summary command:
return list
One of the stored results after an estimation command is the function e(sample). e(sample) returns a one column matrix. If an observation is used in the estimation command it will have a value of 1 in this matrix. If it is not used it will have a value of 0.
Remember that the “stored” results are in temp files. They will disappear the next time you run another estimation command.
The Steps
So how do I use the same sample for all my models? Follow these steps.
Using the regression example on mental health I determine which model has the fewest observations. In this case it was model four.
I rerun the model:
regress MCS weeks_unemployed i.marital_status kids_in_house religious_attend income
Next I use the generate command to create a new variable whose value is 1 if the observation was in the model and 0 if the observation was not. I will name the new variable “in_model_4”.
gen in_model_4 = e(sample)
Now I will re-run my four regressions and include only the observations that were used in model 4. I will store the models using different names so that I can compare them to the original models.
My commands to run the models are:
regress MCS weeks_unemployed i.marital_status if in_model_4==1
estimates store model_1a
regress MCS weeks_unemployed i.marital_status kids_in_house if in_model_4==1
estimates store model_2a
regress MCS weeks_unemployed i.marital_status kids_in_house religious_attend if in_model_4==1
estimates store model_3a
regress MCS weeks_unemployed i.marital_status kids_in_house religious_attend income if in_model_4==1
estimates store model_4a
Note: I could use the code if in_model_4 instead of if in_model_4==1. Stata interprets dummy variables as 0 = false, 1 = true.
Here are the results comparing the original models (eg. Model_1) versus the models using the same sample (eg. Model_1a):
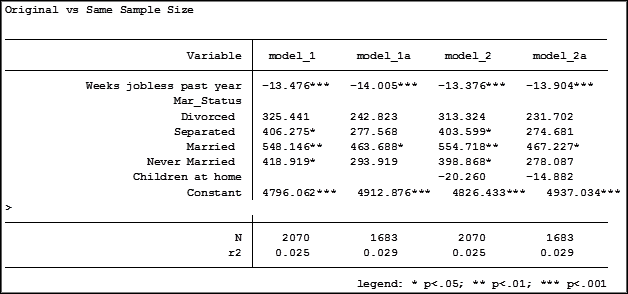
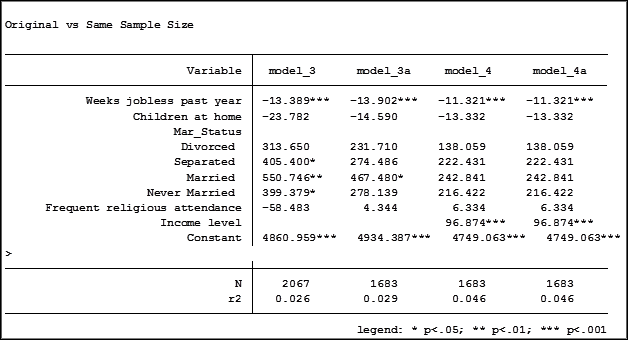
Comparing the original models 3 and 4 one would have assumed that the predictor variable “Income level” significantly impacted the coefficient of “Frequent religious attendance”. Its coefficient changed from -58.48 in model 3 to 6.33 in model 4.
That would have been the wrong assumption. That change is coefficient was not so much about any effect of the variable itself, but about the way it causes the sample to change via listwise deletion. Using the same sample, the change in the coefficient between the two models is very small, moving from 4 to 6.
Jeff Meyer is a statistical consultant with The Analysis Factor, a stats mentor for Statistically Speaking membership, and a workshop instructor. Read more about Jeff here.