Most Multiple Imputation methods assume multivariate normality, so a common question is how to impute missing values from categorical variables.
Paul Allison, one of my favorite authors of statistical information for researchers, did a study that showed that the most common method actually gives worse results that listwise deletion. (Did I mention I’ve used it myself?) (more…)
In choosing an approach to missing data, there are a number of things to consider. But you need to keep in mind what you’re aiming for before you can even consider which approach to take.
There are three criteria we’re aiming for with any missing data technique:
1. Unbiased parameter estimates: Whether you’re estimating means, regressions, or odds ratios, you want your parameter estimates to be accurate representations of the actual population parameters. In statistical terms, that means the estimates should be unbiased. If all the (more…)
I’m sure I don’t need to explain to you all the problems that occur as a result of missing data. Anyone who has dealt with missing data—that means everyone who has ever worked with real data—knows about the loss of power and sample size, and the potential bias in your data that comes with listwise deletion.
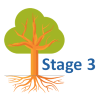
Listwise deletion is the default method for dealing with missing data in most statistical software packages. It simply means excluding from the analysis any cases with data missing on any variables involved in the analysis.
A very simple, and in many ways appealing, method devised to (more…)
There are many ways to approach missing data. The most common, I
believe, is to ignore it. But making no choice means that your statistical software is choosing for you.
Most of the time, your software is choosing listwise deletion. Listwise deletion may or may not be a bad choice, depending on why and how much data are missing.
Another common approach among those who are paying attention is imputation. Imputation simply means replacing the missing values with an estimate, then analyzing the full data set as if the imputed values were actual observed values.
How do you choose that estimate? The following are common methods: (more…)