If you’ve been doing data analysis for long, you’ve probably had the ‘AHA’ moment where you realized statistical practice is a craft and not just a science. As with any craft, there are best practices that will save you a
lot of pain and suffering and elevate the quality of your work. And yet, it’s likely that no one may have taught you these. I know I never had a class on this. (more…)
Every time you analyze data, you start with a research question and end with communicating an answer. But in between those start and end points are twelve other steps. I call this the Data Analysis Pathway. It’s a framework I put together years ago, inspired by a client who kept getting stuck in Weed #1. But I’ve honed it over the years of assisting thousands of researchers with their analysis.
(more…)
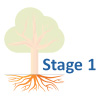
Data Cleaning is a critically important part of any data analysis. Without properly prepared data, the analysis will yield inaccurate results. Correcting errors later in the analysis adds to the time, effort, and cost of the project.
(more…)
It’s easy to think that if you just knew statistics better, data analysis wouldn’t be so hard.
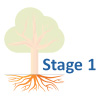
It’s true that more statistical knowledge is always helpful. But I’ve found that statistical knowledge is only part of the story.
Another key part is developing data analysis skills. These skills apply to all analyses. It doesn’t matter which statistical method or software you’re using. So even if you never need any statistical analysis harder than a t-test, developing these skills will make your job easier.
(more…)
Survey questions are often structured without regard for ease of use within a statistical model.
Take for example a survey done by the Centers for Disease Control (CDC) regarding child births in the U.S. One of the variables in the data set is “interval since last pregnancy”. Here is a histogram of the results.
(more…)
You probably learned about the four levels of measurement in your very first statistics class: nominal, ordinal, interval, and ratio.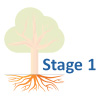
Knowing the level of measurement of a variable is crucial when working out how to analyze the variable. Failing to correctly match the statistical method to a variable’s level of measurement leads either to nonsense or to misleading results.
But the simple framework of the four levels is too simplistic in most real-world data analysis situations.
(more…)