I just discovered something in SPSS GLM that I never knew.
When you have an interaction in the model, the order you put terms into the Model statement affects which parameters SPSS gives you.
The default in SPSS is to automatically create interaction terms among all the categorical predictors. But if you want fewer than all those interactions, or if you want to put in an interaction involving a continuous variable, you need to choose Model–>Custom Model.
In the specific example of an interaction between a categorical and continuous variable, to interpret this interaction you need to output Regression Coefficients. Do this by choosing Options–>Regression Parameter Estimates.
If you put the main effects into the model first, followed by interactions, you will find the usual output–the regression coefficients (column B) for the continuous variable is the slope for the reference group. The coefficients for the interactions in the other categories tell you the difference between the slope for that category and the slope for the reference group. The coefficient for the reference group here in the interaction is 0.
What I was surprised to find is that if the interactions are put into the model first, you don’t get that.
Instead, the coefficients for the interaction of each category is the actual slope for that group, NOT the difference.
This is actually quite useful–it can save a bit of calculating and now you have a p-value for whether each slope is different from 0. However, it also means you have to be cautious and make sure you realize what each parameter estimate is actually estimating.
I first encountered the Great Likert Data Debate in 1992 in my first statistics class in my psychology graduate program.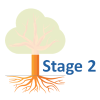
My stats professor was a brilliant mathematical psychologist and taught the class unlike any psychology grad class I’ve ever seen since. Rather than learn ANOVA in SPSS, we derived the Method of Moments using Matlab. While I didn’t understand half of what was going on, this class roused my curiosity and led me to take more theoretical statistics classes. The rest is history.
A large section of the class was dedicated to the fact that Likert data was not interval and therefore not appropriate for statistics that assume normality such as ANOVA and regression. This was news to me. Meanwhile, most of the rest of the field either ignored or debated this assertion.
16 years later, the debate continues. A nice discussion of the debate is found on the Research Methodology blog by Hisham bin Md-Basir. It’s a nice blog with thoughtful entries that summarize methodological articles in the social and design sciences.
To be fair, though, this blog entry summarizes an article on the “Likert scales are not interval” side of the debate. For a balanced listing of references, see Can Likert Scale Data Ever Be Continuous?
I recently had this question in consulting:
I’ve got 12 out of 645 cases with Mahalanobis’s Distances above the critical value, so I removed them and reran the analysis, only to find that another 10 cases were now outside the value. I removed these, and another 10 appeared, and so on until I have removed over 100 cases from my analysis! Surely this can’t be right!?! Do you know any way around this? It is really slowing down my analysis and I have no idea how to sort this out!!
And this was my response:
I wrote an article about dropping outliers. As you’ll see, you can’t just drop outliers without a REALLY good reason. Being influential is not in itself a good enough reason to drop data.
Should you drop outliers? Outliers are one of those statistical issues that everyone knows about, but most people aren’t sure how to deal with. Most parametric statistics, like means, standard deviations, and correlations, and every statistic based on these, are highly sensitive to outliers.
And since the assumptions of common statistical procedures, like linear regression and ANOVA, are also based on these statistics, outliers can really mess up your analysis.
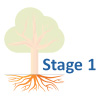
Despite all this, as much as you’d like to, it is NOT acceptable to
(more…)
There are probably many myths about statistics, but there is one that I believe leads to the most frustration in researchers (and students) as they attempt to learn and apply statistics.
The Carpentry Class: A Fable
There was once a man who needed to build a house. He had a big pile of lumber, and he needed a place to live, so building one himself seemed like a good idea.
He realized that he did not have the knowledge and many skills needed to build a house.
So he did what any intelligent, well-educated person would do. He took a course: House Building 101.
There was a lot of new jargon: trusses, plumb walls, 16” on center, cripple studs. It was hard to keep it all straight. It didn’t make sense. Why would anyone ever need a header anyway?
But he made it through with a B+. He learned the basics. The doghouse he built in the lab was pretty straight. He even took another course to make sure he knew enough: Advanced Carpentry.
It was time for the man to build his house. He had his land, his plan, his tools, his sacks of concrete, windows, lumber, and nails.
The first day he started with enthusiasm. He swung his hammer with gusto and nailed his first wall into place. It felt good.
But wait. His house was being built on a hill. The textbook only had flat land. How should he deal with hills?
And this house has a bay window. His doghouse had only double hung windows. Doesn’t a bay window stick out?
And he was not sure which technique to use to make that 145 degree angle in the hall. The courses never mentioned anything but 90 degree angles.
In class, they used circular saws. In order to install the trim he ordered, he needed to use a chop saw and a table saw.
He didn’t realize he was supposed to put in the plumbing before the electric, so he ended up doing a LOT of rewiring when the plumbing wouldn’t fit around his wires.
Even with the plans in front of him, there were so many decisions to make, so many new skills to learn.
And he was supposed to move into the house in 4 months when his lease ran out. He’d never get it done in time. Not on his own.
He sounds like a fool, doesn’t he? No one could build a house after taking even a few courses. Especially not with a deadline.
Building a house requires the knowledge of how walls are constructed, sure. But it also requires the ability to use the tools, and the practical skills to implement the techniques.
We can see that this project was a silly one to tackle, yet all the time we think it’s our fault that we have trouble with statistical analysis after taking a few classes.
The Statistics Myth:
Having knowledge about statistics is the only thing necessary to practice statistics.
This isn’t true.
And it’s not helpful.
Yes, the knowledge is necessary, but it is not sufficient.
Statistics doesn’t make sense to students because it is taught out of context. Most people don’t really learn statistics until they start analyzing data in their own research. Yes, it makes those classes tough. You need to acquire the knowledge before you can truly understand it.
The only way to learn how to build a house is to build one. The only way to learn how to analyze data is to analyze some.
Here’s the thing. Data analysts (and house builders) need practical support as they learn. Yes, both could slug it out on their own, but it takes longer, is more frustrating, and leads to many more mistakes.
None of this is necessary. There can be a happy ending.
Carpenters work alongside a master to learn their craft. I have never heard of a statistician or a thesis advisor who sits next to a novice analyzing data. (Anyone who had an advisor like that should consider themselves lucky). Unlike a novice carpenter, a novice data analyst is not helpful. They can’t even hold the ladder.
More common are advisors who tell their students which statistics classes to take (again, if they’re lucky) then send them off to analyze data. The student can ask questions as they go along if they are not too afraid to admit what they don’t know. And if their advisor is available. And knows the answer.
Really good advisors are not too busy to answer in a timely manner and are willing to admit it if they don’t know the answer.
But most data analysts feel a bit lost. Not just new ones—many experienced researchers never really learned statistical practice very well in the first place. Nearly all researchers face new statistical challenges as their research progresses, and it’s often difficult to find someone knowledgeable enough who is willing to and able explain it.
They are not lost because they are stupid.
They are not lost because statistics is beyond their capabilities.
They are not lost because they didn’t do well in their statistics classes.
They are lost because like carpentry, statistical analysis is an applied skill, a craft.
Acquiring the background knowledge is only one essential part of mastering a craft.
Others include:
- a belief you can do it
- a commitment to best practices
- experience in applying the skills in different situations
- proficiency in using the tools
- a resource library
- ongoing training to learn new skills
- (ideally) a mentor to guide you as you practice.
Think about it. How many skills (dancing, sailing, teaching) have you acquired in your life by only taking a class that gave you background knowledge, but no real experience and no real mentor to coach you?
So if you’re stuck on something in statistics, give yourself a break. You can do this with the right support.
Everything we do at The Analysis Factor is to help you get unstuck. If you’re frustrated, tired, or even scared…there is another way.
If you need help right now, we’ve got your back. Please check out our Statistical Consulting services and our Statistically Speaking membership.