One of the most anxiety-laden questions I get from researchers is whether their analysis is “right.”
I’m always slightly uncomfortable with that word. Often there is no one right analysis.
It’s like finding Mr. or Ms. Right. Most of the time, there is not just one Right. But there are many that are clearly Wrong.
What Makes an Analysis Right?
Luckily, what makes an analysis right is easier to define than what makes a person right for you. It pretty much comes down to two things: whether the assumptions of the statistical method are being met and whether the analysis answers the research question.
Assumptions are very important. A test needs to reflect the measurement scale of the variables, the study design, and issues in the data. A repeated measures study design requires a repeated measures analysis. A binary dependent variable requires a categorical analysis method.
But within those general categories, there are often many analyses that meet assumptions. A logistic regression or a chi-square test both handle a binary dependent variable with a single categorical predictor. But a logistic regression can answer more research questions. It can incorporate covariates, directly test interactions, and calculate predicted probabilities. A chi-square test can do none of these.
So you get different information from different tests. They answer different research questions.
An analysis that is correct from an assumptions point of view is useless if it doesn’t answer the research question. A data set can spawn an endless number of statistical tests that don’t answer the research question. And you can spend an endless number of days running them.
When to Think about the Analysis
The real bummer is it’s not always clear that the analyses aren’t relevant until you write up the research paper.
That’s why writing out the research questions in theoretical and operational terms is the first step of any statistical analysis. It’s absolutely fundamental. And I mean writing them in minute detail. Issues of mediation, interaction, subsetting, control variables, et cetera, should all be blatantly obvious in the research questions.
Thinking about how to analyze the data before collecting the data can help you from hitting a dead end. It can be very obvious, once you think through the details, that the analysis available to you based on the data won’t answer the research question.
Whether the answer is what you expected or not is a different issue.
So when you are concerned about getting an analysis “right,” clearly define the design, variables, and data issues, but most importantly, get explicitly clear about what you want to learn from this analysis.
Once you’ve done this, it’s much easier to find the statistical method that answers the research questions and meets assumptions. Even if you don’t know the right method, you can narrow your search with clear guidance.
Many years ago, when I was teaching in a statistics department, I had my first consulting gig. Two psychology researchers didn’t know how to analyze their paired rank data. Unfortunately, I didn’t either. I asked a number of statistics colleagues (who didn’t know either), then finally borrowed a nonparametrics book. The answer was right there. (If you’re curious, it was a Friedman test.)
But the bigger lesson for me was the importance of a good reference library. No matter how much statistical training and experience you have, you won’t remember every detail about every statistical test. And you don’t need to. You just need to have access to the information and be able to understand it.
My statistics library consists of a collection of books, software manuals, articles, and web sites. Yet even in the age of Google, the heart of my library is still books. I use Google when I need to look something up, but it’s often not as quick as I’d hoped, and I don’t always find the answer. I rely on my collection of good reference books that I KNOW will have the answer I’m looking for (and continually add to it).
Not all statistics books are equally helpful in every situation. I divide books into four categories– Reference Books, Software Books, Applied Statistics Books, and data analysis books. My library has all four, and yours should too, if data analysis is something you’ll be doing long-term. I’ve included examples for running logistic regression in SAS, so you can compare the four types.
1. Reference Books are often text books. They are filled with formulas, theory, and exercises, as well as explanations. As a data analyst, not a student, you can skip most of it and go right for the explanations or formula you need. While I find most text books aren’t useful for learning HOW to do a new statistical method on your own, they are great references for already-familiar methods.
While I have a few favorites, the best one is often the one you already own and are familiar with, i.e. the textbooks you used in your stats classes. Hopefully, you didn’t sell back your stats text books (or worse, have the post office lose them in your cross-country move, like I did).
Example: Alan Agresti’s Categorical Data Analysis.
2. Statistical Software Books focus on using a software package. They tend to be general, often starting from the beginning, and cover everything from entering and manipulating data to advanced statistical techniques. This is the type of book to use when learning a new package or area of a package. They don’t, however, usually tell you much about the actual statistics–what it means, why to use it, or when different options make sense. And these are not manuals–they are usually written by users of the software, and are much better for learning a software program. (I think of learning a software program like learning French from a French dictionary–not so good).
Example: Ron Cody & Jeffrey Smith’s Applied Statistics and the SAS Programming Language
3. Applied Statistics Books are written for researchers. The focus is not on the formulas, as text books are, but on meaning and use of the statistics. Good applied statistics books are fabulous for learning a new technique when you don’t have time for a semester-length class, but you will have to have a reasonably strong statistical background to read or use them well. They aren’t for beginners. The nice thing about applied statistics books is they are not tied to any piece of software, so they’re useful to anyone. That is also their limitation, though–they won’t guide you through the actual analysis in your package.
Example: Scott Menard’s Applied Logistic Regression Analysis
4. Statistical Analysis Books are a hybrid between applied statistics and statistical software books. They explain both the steps to the software AND what it all means. There aren’t many of these, but many of the ones that exist are great. The only problem is they are often published by the software companies, so each one only exists for one software package. If it’s not the one you use, they’re less useful. But they are often great anyway as Applied Statistics books.
Example: Paul Allison’s Logistic Regression using the SAS System: Theory and Application
If you are without reference books you like, buy them used. Unlike students, you don’t need the latest edition. Most areas of statistics don’t change that much. Linear regression isn’t getting new assumptions, and factor analysis isn’t getting new rotations. Unless it’s in an area of statistics that is still developing, like multilevel modeling and missing data, you’re pretty safe with a 10 year old version.
And it does help to buy them. Use your institution’s library to supplement your personal library. Even if it’s great, getting to that library is an extra barrier, and waiting a few weeks for the recall or interlibrary loan is sometimes too long.
I have bought used textbooks for $10. Menard’s book, and all of the excellent Sage series, are only $17, new. So it doesn’t have to cost a fortune to build a library. Even so, paying $70 for a book is sometimes completely worth it. Having the information you need will save you hours, or even days of work. How much is your time and energy worth? If you plan to do data analysis long term, invest a little each year in statistical reference books.
The full list of all four types of books Karen recommends is on The Analysis Factor Bookshelf page.
If you know of any other great books we should recommend, comment below. I’m always looking for good books to recommend.
You don’t rely on only SPSS menus to run your analysis, right? (Please, please tell me you don’t).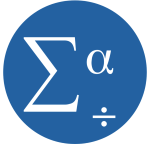
There’s really nothing wrong with using the menus. It’s a great way to get started using SPSS and it saves you the hassle of remembering all that code.
But there are some really, really good reasons to use the syntax as well.
1. Efficiency
If you’re figuring out the best model and have to refine which predictors to include, running the same descriptive statistics on a bunch of variables, or defining the missing values for all 286 variable in the data set, you’re essentially running the same analysis over and over.
Picking your way through the menus gets old fast. In syntax, you just copy and paste and change or add variables names.
A trick I use is to run through the menus for one variable, paste the code, then add the other 285. You can even copy the names out of the Variable View and paste them into the code. Very easy.
2. Memory
I know that while you’re immersed in your data analysis, you can’t imagine you won’t always remember every step you did.
But you will. And sooner than you think.
Syntax gives you a “paper” trail of what you did, so you don’t have to remember. If you’re in a regulated industry, you know why you need this trail. But anyone who needs to defend their research needs it.
3. Communication
When your advisor, coauthor, colleague, statistical consultant, or Reviewer #2 asks you which options you used in your analysis or exactly how you recoded that variable, you can clearly communicate it by showing the syntax. Much harder to explain with menu options.
When I hold a workshop or run an analysis for a client, I always use syntax. I send it to them to peruse, tweak, adapt, or admire. It’s really the only way for me to show them exactly what I did and how to do it.
If your client, advisor, or colleague doesn’t know how to read the syntax, that’s okay. Because you have a clear answer of what you did, you can explain it.
4. Efficiency again
When the data set gets updated, or a reviewer (or your advisor, coauthor, colleague, or statistical consultant) asks you to add another predictor to a model, it’s a simple matter to edit and rerun a syntax program.
In menus, you have to start all over. Hopefully you’ll remember exactly which options you chose last time and/or exactly how you made every small decision in your data analysis (see #2: Memory).
5. Control
There are some SPSS options that are available in syntax, but not in the menus.
And others that just aren’t what they seem in the menus.
The menus for the Mixed procedure are about the most unintuitive I’ve ever seen. But the syntax for Mixed is really logical and straightforward. And it’s very much like the GLM syntax (UNIANOVA), so if you’re familiar with GLM, learning Mixed is a simple extension.
Bonus Reason to use SPSS Syntax: Cleanliness
Luckily, SPSS makes it exceedingly easy to create syntax. If you’re more comfortable with menus, run it in menus the first time, then hit PASTE instead of OK. SPSS will automatically create the syntax for you, which you can alter at will. So you don’t have to remember every programming convention.
When refining a model, I often run through menus and paste it. Then I alter the syntax to find the best-fitting model.
At this point, the output is a mess, filled with so many models I can barely keep them straight. Once I’ve figured out the model that fits best, I delete the entire output, then rerun the syntax for only the best model. Nice, clean output.
The Take-away: Reproducibility
What this all really comes down to is your ability to confidently, easily, and accurately reproduce your analysis. When you rely on menus, you are relying on your own memory to reproduce. There are too many decisions, judgments, and too many places to make easy mistakes without noticing it to ever be able to rely totally on your memory.
The tools are there to make this easy. Use them.
After nearly twenty years of helping researchers hone their statistical skills to become better data analysts, I’ve had a few insights about what that process looks like.
The one thing you don’t need to become a great data analyst is some innate statistical genius. That kind of fixed mindset will undermine the growth in your statistical skills.
So to start your journey become a skilled and confident statistical analyst, you need: (more…)
My 8 year-old son got a Rubik’s cube in his Christmas stocking this year.
I had gotten one as a birthday present when I was about 10. It was at the height of the craze and I was so excited.
I distinctly remember bursting into tears when I discovered that my little sister sneaked playing with it, and messed it up the day I got it. I knew I would mess it up to an unsolvable point soon myself, but I was still relishing the fun of creating patterns in the 9 squares, then getting it back to 6 sides of single-colored perfection. (I loved patterns even then). (more…)
I was recently asked this question about Chi-square tests. This question comes up a lot, so I thought I’d share my answer.
I have to compare two sets of categorical data in a 2×4 table. I cannot run the chi-square test because most of the cells contain values less than five and a couple of them contain values of 0. Is there any other test that I could use that overcomes the limitations of chi-square?
And here is my answer: (more…)