Just last week, a colleague mentioned that while he does a lot of study design these days, he no longer does much data analysis.
His main reason was that 80% of the work in data analysis is preparing the data for analysis. Data preparation is s-l-o-w and he found that few colleagues and clients understood this.
Consequently, he was running into expectations that he should analyze a raw data set in an hour or so.
You know, by clicking a few buttons.
I see this as well with researchers new to data analysis. While they know it will take longer than an hour, they still have unrealistic expectations about how long it takes.
So I am here to tell you, the time-consuming part is preparing the data. Weeks or months is a realistic time frame. Hours is not.
(Feel free to send this to your colleagues who want instant results.)
There are three parts to preparing data: cleaning it, creating necessary variables, and formatting all variables.
Data Cleaning
Data cleaning means finding and eliminating errors in the data. How you approach it depends on how large the data set is, but the kinds of things you’re looking for are:
- Impossible or otherwise incorrect values for specific variables
- Cases in the data who met exclusion criteria and shouldn’t be in the study
- Duplicate cases
- Missing data and outliers (don’t delete all outliers, but you may need to investigate to see if one is an error)
- Skip-pattern or logic breakdowns
- Making sure that the same value of string variables is always written the same way (male ≠ Male in most statistical software).
You can’t avoid data cleaning and it always takes a while, but there are ways to make it more efficient. For example, rather than search through the data set for impossible values, print a table of data values outside a normal range, along with subject ids.
This is where learning how to code in your statistical software of choice really helps. You’ll need to subset your data using IF statements to find those impossible values.
But if your data set is anything but small, you can also save yourself a lot of time, code, and errors by incorporating efficiencies like loops and macros so that you can perform some of these checks on many variables at once.
Creating New Variables
Once the data are free of errors, you need to set up the variables that will directly answer your research questions.
It’s a rare data set in which every variable you need is measured directly.
So you may need to do a lot of recoding and computing of variables.
Examples include:
And of course, part of creating each new variable is double-checking that it worked correctly.
Formatting Variables
Both original and newly created variables need to be formatted correctly for two reasons:
First, so your software works with them correctly. Failing to format a missing value code or a dummy variable correctly will have major consequences for your data analysis.
Second, it’s much faster to run the analyses and interpret results if you don’t have to keep looking up which variable Q156 is.
Examples include:
- Setting all missing data codes so missing data are treated as such
- Formatting date variables as dates, numerical variables as numbers, etc.
- Labeling all variables and categorical values so you don’t have to keep looking them up.
All three of these steps require a solid knowledge of how to manage data in your statistical software. Each one approaches them a little differently.
It’s also very important to keep track of and be able to easily redo all your steps. Always assume you’ll have to redo something. So use (or record) syntax, not only menus.
Do I really need to learn R?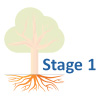
Someone asked me this recently.
Many R advocates would absolutely say yes to everyone who asks.
I don’t.
(I actually gave her a pretty long answer, summarized here).
It depends on what kind of work you do and the context in which you’re working.
I can say that R is (more…)
In the world of data analysis, there’s not always one clearly appropriate statistical analysis for every research question.
There are so many issues to take into account. They include the research question to be answered, the measurement of the variables, the study design, data limitations and issues, the audience, practical constraints like software availability, and the purpose of the data analysis.
So what do you do when a reviewer rejects your choice of data analysis? This reviewer can be your boss, your dissertation committee, a co-author, or journal reviewer or editor.
What do you do?
There are ultimately only two choices: You can redo the analysis their way. Or you can fight for your analysis. How do you choose?
The one absolute in this choice is that you have to honor the integrity of your data analysis and yourself.
Do not be persuaded to do an analysis that will produce inaccurate or misleading results, especially when readers will actually make decisions based on these results. (If no one will ever read your report, this is less crucial).
But even within that absolute, there are often choices. Keep in mind the two goals in data analysis:
- The analysis needs to accurately reflect the limits of the design and the data, while still answering the research question.
- The analysis needs to communicate the results to the audience.
When to fight for your analysis
So first and foremost, if your reviewer is asking you to do an analysis that does not appropriately take into account the design or the variables, you need to fight.
For example, a few years ago I worked with a researcher who had a study with repeated measurements on the same individuals. It had a small sample size and an unequal number of observations on each individual.
It was clear that to take into account the design and the unbalanced data, the appropriate analysis was a linear mixed model.
The researcher’s co-author questioned the use of the linear mixed model, mainly because he wasn’t familiar with it. He thought the researcher was attempting something fishy. His suggestion was to use an ad hoc technique of averaging over the multiple observations for each subject.
This was a situation where fighting was worth it.
Unnecessarily simplifying the analysis to please people who were unfamiliar with an appropriate method was not an option. The simpler model would have violated assumptions.
This was particularly important because the research was being submitted to a high-level journal.
So it was the researcher’s job to educate not only his coauthor, but the readers, in the form of explaining the analysis and its advantages, with citations, right in the paper.
When to Jump through Hoops
In contrast, sometimes the reviewer is not really asking for a completely different analysis. They just want a different way of running the same analysis or reporting different specific statistics.
For example a simple confirmatory factor analysis can be run in standard statistical software like SAS, SPSS, or Stata using a factor analysis command. Or it can be run it in structural equation modeling software like Amos or MPlus or using an SEM command in standard software.
The analysis is essentially the same, but the two types of software will report different statistics.
If your committee members are familiar with structural equation modeling, they probably want to see the type of statistics that structural equation modeling software will report. Running it this way has advantages.
These include overall model fit statistics like RMSEA or model chi-squares.
This is a situation where it may be easier, and produces no ill-effects, to jump through the hoop.
Running the analysis in the software they prefer won’t violate any assumptions or produce inaccurate results. This assumes you have access to that software and know how to use it.
If the reviewer can stop your research in its tracks, it may be worth it to rerun the analysis to get the statistics they want to see reported.
You do have to decide whether the cost of jumping through the hoop, in terms of time, money, and emotional energy, is worth it.
If the request is relatively minor, it usually is. If it’s a matter of rerunning every analysis you’ve done to indulge a committee member’s pickiness, it may be worth standing up for yourself and your analysis.
When you can’t talk to the reviewer
When you’re dealing with anonymous reviewers, the situation can get sticky. After all, you cannot ask them to clarify their concerns. And you have limited opportunities to explain the reasons for choosing your analysis.
It may be harder to discern if they are being overly picky, don’t understand the statistics themselves, or have a valid point.
If you choose to stand up for yourself, be well armed. Research the issue until you are absolutely confident in your approach (or until you’re convinced that you were missing something).
A few hours in the library or talking with a trusted expert is never a wasted investment. Compare that to running an unpublishable analysis to please a committee member or coauthor.
Often, the problem is actually not in the analysis you did, but in the way you explained it. It’s your job to explain why the analysis is appropriate and, if it’s unfamiliar to readers, what it does.
Rewrite that section, making it very clear. Ask someone to review it. Cite other research that uses or explains that statistical method.
Whatever you choose, be confident that you made the right decision, then move on.
Knowing the right statistical analysis to use in any data situation, knowing how to run it, and being able to understand the output are all really important skills for statistical analysis. Really important.
But they’re not the only ones.
Another is having a system in place to keep track of the analyses. This is especially important if you have any collaborators (or a statistical consultant!) you’ll be sharing your results with. You may already have an effective work flow, but if you don’t, here are some strategies I use. I hope they’re helpful to you.
1. Always use Syntax Code
All the statistical software packages have come up with some sort of easy-to-use, menu-based approach. And as long as you know what you’re doing, there is nothing wrong with using the menus. While I’m familiar enough with SAS code to just write it, I use menus all the time in SPSS.
But even if you use the menus, paste the syntax for everything you do. There are many reasons for using syntax, but the main one is documentation. Whether you need to communicate to someone else or just remember what you did, syntax is the only way to keep track. (And even though, in the midst of analyses, you believe you’ll remember how you did something, a week and 40 models later, I promise you won’t. I’ve been there too many times. And it really hurts when you can’t replicate something).
In SPSS, there are two things you can do to make this seamlessly easy. First, instead of hitting OK, hit Paste. Second, make sure syntax shows up on the output. This is the default in later versions, but you can turn in on in Edit–>Options–>Viewer. Make sure “Display Commands in Log” and “Log” are both checked. (Note: the menus may differ slightly across versions).
2. If your data set is large, create smaller data sets that are relevant to each set of analyses.
First, all statistical software needs to read the entire data set to do many analyses and data manipulation. Since that same software is often a memory hog, running anything on a large data set will s-l-o-w down processing. A lot.
Second, it’s just clutter. It’s harder to find the variables you need if you have an extra 400 variables in the data set.
3. Instead of just opening a data set manually, use commands in your syntax code to open data sets.
Why? Unless you are committing the cardinal sin of overwriting your original data as you create new variables, you have multiple versions of your data set. Having the data set listed right at the top of the analysis commands makes it crystal clear which version of the data you analyzed.
I know you remember today that your variable labeled Mar4cat means marital status in 4 categories and that 0 indicates ‘never married.’ It’s so logical, right? Well, it’s not obvious to your collaborators and it won’t be obvious to you in two years, when you try to re-analyze the data after a reviewer doesn’t like your approach.
Even if you have a separate code book, why not put it right in the data? It makes the output so much easier to read, and you don’t have to worry about losing the code book. It may feel like more work upfront, but it will save time in the long run.
5. Put data manipulation, descriptive analyses, and models in separate syntax files
When I do data analysis, I follow my Steps approach, which means first I create all the relevant variables, then run univariate and bivariate statistics, then initial models, and finally hone the models.
And I’ve found that if I keep each of these steps in separate program files, it makes it much easier to keep track of everything. If you’re creating new variables in the middle of analyses, it’s going to be harder to find the code so you can remember exactly how you created that variable.
6. As you run different versions of models, label them with model numbers
When you’re building models, you’ll often have a progression of different versions. Especially when I have to communicate with a collaborator, I’ve found it invaluable to number these models in my code and print that model number on the output. It makes a huge difference in keeping track of nine different models.
7. As you go along with different analyses, keep your syntax clean, even if the output is a mess.
Data analysis is a bit of an iterative process. You try something, discover errors, realize that variable didn’t work, and try something else. Yes, base it on theory and have a clear analysis plan, but even so, the first analyses you run won’t be your last.
Especially if you make mistakes as you go along (as I inevitably do), your output gets pretty littered with output you don’t want to keep. You could clean it up as you go along, but I find that’s inefficient. Instead, I try to keep my code clean, with only the error-free analyses that I ultimately want to use. It lets me try whatever I need to without worry. Then at the end, I delete the entire output and just rerun all code.
One caveat here: You may not want to go this approach if you have VERY computing intensive analyses, like a generalized linear mixed model with crossed random effects on a large data set. If your code takes more than 20 minutes to run, this won’t be more efficient.
8. Use titles and comments liberally
I’m sure you’ve heard before that you should use lots of comments in your syntax code. But use titles too. Both SAS and SPSS have title commands that allow titles to be printed right on the output. This is especially helpful for naming and numbering all those models in #6.
9. Name output, log, and programs the same
Since you’ve split your programs into separate files for data manipulations, descriptives, initial models, etc. you’re going to end up with a lot of files. What I do is name each output the same name as the program file. (And if I’m in SAS, the log too-yes, save the log).
Yes, that means making sure you have a separate output for each section. While it may seem like extra work, it can make looking at each output less overwhelming for anyone you’re sharing it with.
One of the most anxiety-laden questions I get from researchers is whether their analysis is “right.”
I’m always slightly uncomfortable with that word. Often there is no one right analysis.
It’s like finding Mr. or Ms. Right. Most of the time, there is not just one Right. But there are many that are clearly Wrong.
What Makes an Analysis Right?
Luckily, what makes an analysis right is easier to define than what makes a person right for you. It pretty much comes down to two things: whether the assumptions of the statistical method are being met and whether the analysis answers the research question.
Assumptions are very important. A test needs to reflect the measurement scale of the variables, the study design, and issues in the data. A repeated measures study design requires a repeated measures analysis. A binary dependent variable requires a categorical analysis method.
But within those general categories, there are often many analyses that meet assumptions. A logistic regression or a chi-square test both handle a binary dependent variable with a single categorical predictor. But a logistic regression can answer more research questions. It can incorporate covariates, directly test interactions, and calculate predicted probabilities. A chi-square test can do none of these.
So you get different information from different tests. They answer different research questions.
An analysis that is correct from an assumptions point of view is useless if it doesn’t answer the research question. A data set can spawn an endless number of statistical tests that don’t answer the research question. And you can spend an endless number of days running them.
When to Think about the Analysis
The real bummer is it’s not always clear that the analyses aren’t relevant until you write up the research paper.
That’s why writing out the research questions in theoretical and operational terms is the first step of any statistical analysis. It’s absolutely fundamental. And I mean writing them in minute detail. Issues of mediation, interaction, subsetting, control variables, et cetera, should all be blatantly obvious in the research questions.
Thinking about how to analyze the data before collecting the data can help you from hitting a dead end. It can be very obvious, once you think through the details, that the analysis available to you based on the data won’t answer the research question.
Whether the answer is what you expected or not is a different issue.
So when you are concerned about getting an analysis “right,” clearly define the design, variables, and data issues, but most importantly, get explicitly clear about what you want to learn from this analysis.
Once you’ve done this, it’s much easier to find the statistical method that answers the research questions and meets assumptions. Even if you don’t know the right method, you can narrow your search with clear guidance.
Many years ago, when I was teaching in a statistics department, I had my first consulting gig. Two psychology researchers didn’t know how to analyze their paired rank data. Unfortunately, I didn’t either. I asked a number of statistics colleagues (who didn’t know either), then finally borrowed a nonparametrics book. The answer was right there. (If you’re curious, it was a Friedman test.)
But the bigger lesson for me was the importance of a good reference library. No matter how much statistical training and experience you have, you won’t remember every detail about every statistical test. And you don’t need to. You just need to have access to the information and be able to understand it.
My statistics library consists of a collection of books, software manuals, articles, and web sites. Yet even in the age of Google, the heart of my library is still books. I use Google when I need to look something up, but it’s often not as quick as I’d hoped, and I don’t always find the answer. I rely on my collection of good reference books that I KNOW will have the answer I’m looking for (and continually add to it).
Not all statistics books are equally helpful in every situation. I divide books into four categories– Reference Books, Software Books, Applied Statistics Books, and data analysis books. My library has all four, and yours should too, if data analysis is something you’ll be doing long-term. I’ve included examples for running logistic regression in SAS, so you can compare the four types.
1. Reference Books are often text books. They are filled with formulas, theory, and exercises, as well as explanations. As a data analyst, not a student, you can skip most of it and go right for the explanations or formula you need. While I find most text books aren’t useful for learning HOW to do a new statistical method on your own, they are great references for already-familiar methods.
While I have a few favorites, the best one is often the one you already own and are familiar with, i.e. the textbooks you used in your stats classes. Hopefully, you didn’t sell back your stats text books (or worse, have the post office lose them in your cross-country move, like I did).
Example: Alan Agresti’s Categorical Data Analysis.
2. Statistical Software Books focus on using a software package. They tend to be general, often starting from the beginning, and cover everything from entering and manipulating data to advanced statistical techniques. This is the type of book to use when learning a new package or area of a package. They don’t, however, usually tell you much about the actual statistics–what it means, why to use it, or when different options make sense. And these are not manuals–they are usually written by users of the software, and are much better for learning a software program. (I think of learning a software program like learning French from a French dictionary–not so good).
Example: Ron Cody & Jeffrey Smith’s Applied Statistics and the SAS Programming Language
3. Applied Statistics Books are written for researchers. The focus is not on the formulas, as text books are, but on meaning and use of the statistics. Good applied statistics books are fabulous for learning a new technique when you don’t have time for a semester-length class, but you will have to have a reasonably strong statistical background to read or use them well. They aren’t for beginners. The nice thing about applied statistics books is they are not tied to any piece of software, so they’re useful to anyone. That is also their limitation, though–they won’t guide you through the actual analysis in your package.
Example: Scott Menard’s Applied Logistic Regression Analysis
4. Statistical Analysis Books are a hybrid between applied statistics and statistical software books. They explain both the steps to the software AND what it all means. There aren’t many of these, but many of the ones that exist are great. The only problem is they are often published by the software companies, so each one only exists for one software package. If it’s not the one you use, they’re less useful. But they are often great anyway as Applied Statistics books.
Example: Paul Allison’s Logistic Regression using the SAS System: Theory and Application
If you are without reference books you like, buy them used. Unlike students, you don’t need the latest edition. Most areas of statistics don’t change that much. Linear regression isn’t getting new assumptions, and factor analysis isn’t getting new rotations. Unless it’s in an area of statistics that is still developing, like multilevel modeling and missing data, you’re pretty safe with a 10 year old version.
And it does help to buy them. Use your institution’s library to supplement your personal library. Even if it’s great, getting to that library is an extra barrier, and waiting a few weeks for the recall or interlibrary loan is sometimes too long.
I have bought used textbooks for $10. Menard’s book, and all of the excellent Sage series, are only $17, new. So it doesn’t have to cost a fortune to build a library. Even so, paying $70 for a book is sometimes completely worth it. Having the information you need will save you hours, or even days of work. How much is your time and energy worth? If you plan to do data analysis long term, invest a little each year in statistical reference books.
The full list of all four types of books Karen recommends is on The Analysis Factor Bookshelf page.
If you know of any other great books we should recommend, comment below. I’m always looking for good books to recommend.