Tell me if you can relate to this: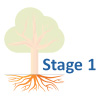
You love your field of study, you enjoy asking the big questions and discovering answers. But, when it comes to data analysis and statistics you get a little bogged down. You might even feel a bit lost sometimes.
And that is hard to admit.
Because after all, you are supposed to be the expert. Right?
Learning Statistics is Hard but We Make it a Lot Easier
The thing is, statistics is a field unto itself. But as a researcher, you need to be adept with statistics even though you may only have very basic training in this field. In essence, you learned statistics — a new language — out of context. You had no real immersion experience to practice this language. You had few opportunities to apply the strange new terms and concepts as you learned them.
At the Analysis Factor, we understand the pain of learning and doing statistics. We have been in the trenches with hundreds of researchers like you across many fields of study. Everyone is struggling to grow their statistical, data analysis, and software skills.
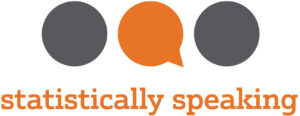
In Statistically Speaking we support and guide you as you learn — every step of the way. We know where to start, where to go next, and next, and next.
We know that your field and research question(s) determine the type of data and complexity of statistical analyses you will choose. And we know that everyone shows up in a different place, and needs different things to help them get where they need to go.
So we have created a treasure trove of resources on hundreds of topics — from data cleaning and research design to logistic regression and structural equation modeling.
And to keep it all about you, we have created a customizable learning platform, one where you make a plan for your own unique journey. We have crafted a series of comprehensive Maps, curated guides on essential topics at each Stage of mastery, offering you a structured pathway through the maze of statistical knowledge.
You create the plan you need, and choose the maps you need to do your research.
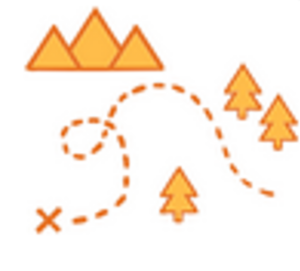
Maps
At The Analysis Factor, we classify the statistical content and skills into 4 Stages to help you decide where to begin your learning journey. In Statistically Speaking, the Maps are categorized into these Stages.
Here are just a few examples:
Stage 1: Fundamentals
-
Preparing Data: Understanding the fundamental steps in data preparation, from cleaning and transforming to structuring datasets for analysis.
-
Bivariate Statistics: Grasping the basics of relationships between two variables, laying the groundwork for more complex analyses.
Stage 2: Linear Models
-
Graphing: Learning visualization techniques to represent data and derive meaningful insights.
-
Introduction to Regression: Unraveling the fundamentals of regression analysis, a cornerstone of statistical modeling.
-
Interpreting Results: Developing the skill to interpret statistical results and draw valid conclusions from analyses.
Stage 3: Extensions of Linear Models
-
Count Models: Exploring specialized models for count data analysis, understanding their application and nuances.
-
Logistic Regression: Diving into binary outcome analysis, understanding probabilities, and logistic models.
-
Factor Analysis: Delving into multivariate analysis, understanding latent variables and their relationships.
Stage 4: Advanced Models
-
GLMM: Embracing the complexity of generalized linear mixed models, integrating fixed and random effects.
-
SEM: Venturing into structural equation modeling, exploring complex relationships among variables.
-
Survival Analysis: Understanding time-to-event data, its application in various fields, and survival modeling techniques.
By mapping out the key content and skills you want to learn at each Stage, you’ll gain a clearer understanding of the vast statistical landscape and feel empowered to take on the learning journey ahead.
So, what are you waiting for? Members, head on over to explore the Maps in Statistically Speaking.
And if you are not yet a member, you can sign-up for our waitlist to join Statistically Speaking. We would love to meet you, learn about your research, and help you get started on your statistical learning adventure.
One of those tricky, but necessary, concepts in statistics is the difference between crossed and nested factors.
As a reminder, a factor is any categorical independent variable. In experiments, or any randomized designs, these factors are often manipulated. Experimental manipulations (like Treatment vs. Control) are factors.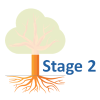
Observational categorical predictors, such as gender, time point, poverty status, etc., are also factors. Whether the factor is observational or manipulated won’t affect the analysis, but it will affect the conclusions you draw from the results.
(more…)
Standard deviation and standard error are statistical concepts you probably learned well enough in Intro Stats to pass the test. Conceptually, you understand them, yet the difference doesn’t make a whole lot of intuitive sense.
So in this article, let’s explore the difference between the two. We will look at an example, in the hopes of making these concepts more intuitive. You’ll also see why sample size has a big effect on standard error. (more…)
What is the difference between Clustered, Longitudinal, and Repeated Measures Data? You can use mixed models to analyze all of them. But the issues involved and some of the specifications you choose will differ.
Just recently, I came across a nice discussion about these differences in West, Welch, and Galecki’s (2007) excellent book, Linear Mixed Models.
It’s a common question. There is a lot of overlap in both the study design and in how you analyze the data from these designs.
West et al give a very nice summary of the three types. Here’s a paraphrasing of the differences as they explain them:
- In clustered data, the dependent variable is measured once for each subject, but the subjects themselves are somehow grouped (student grouped into classes, for example). There is no ordering to the subjects within the group, so their responses should be equally correlated.
- In repeated measures data, the dependent variable is measured more than once for each subject. Usually, there is some independent variable (often called a within-subject factor) that changes with each measurement.
- In longitudinal data, the dependent variable is measured at several time points for each subject, often over a relatively long period of time.
A Few Observations
West and colleagues also make the following good observations:
1. Dropout is usually not a problem in repeated measures studies, in which all data collection occurs in one sitting. It is a huge issue in longitudinal studies, which usually require multiple contacts with participants for data collection.
2. Longitudinal data can also be clustered. If you follow those students for two years, you have both clustered and longitudinal data. You have to deal with both.
3. It can be hard to distinguish between repeated measures and longitudinal data if the repeated measures occur over time. [My two cents: A pre/post/followup design is a classic example].
4. From an analysis point of view, it doesn’t really matter which one you have. All three are types of hierarchical, nested, or multilevel data. You would analyze them all with some sort of mixed or multilevel analysis. You may of course have extra issues (like dropout) to deal with in some of these.
My Own Observations
I agree with their observations, and I’d like to add a few from my own experience.
1. Repeated measures don’t have to be repeated over time. They can be repeated over space (the right knee gets the control operation and the left knee gets the experimental operation). They can also be repeated over condition (each subject gets both the high and low cognitive load condition. Longitudinal studies are pretty much always over time.
This becomes an issue mainly when you are choosing a covariance structure for the within-subject residuals (as determined by the Repeated statement in SAS’s Proc Mixed or SPSS Mixed). An auto-regressive structure is often needed when some repeated measurements are closer to each other than others (over either time or space). This is not an issue with purely clustered data, since there is no order to the observations within a cluster.
2. Time itself is often an important independent variable in longitudinal studies, but in repeated measures studies, it is usually confounded with some independent variable.
When you’re deciding on an analysis, it’s important to think about the role of time. Time is not important in an experiment, where each measurement is a different condition (with order often randomized). But it’s very important in a study designed to measure changes in a dependent variable over the course of 3 decades.
3. Time may be measured with some proxy like Age or Order. But it’s still really about time.
4. A longitudinal study does not have to be over years. You could be measuring reaction time every second for a minute. In cases like this, dropout isn’t an issue, although time is an important predictor.
5. Consider whether it makes sense to think about time as continuous or categorical. If you have only two time points, even if you have numerical measurements for them, there is no point in treating it as continuous. You need at least three time points to fit a line, but more is always better.
6. Longitudinal data can be analyzed with many statistical methods, including structural equation modeling and survival analysis. You only use multilevel modeling if the dependent variable is measured repeatedly and if the point of the model is to see how it changes (or differs).
Naming a data structure, design, or analysis is most helpful if it is so specific that it defines yours exactly. Your repeated measures analysis may not be like the repeated measures example you’re trying to follow. Rather than trying to name the analysis or the data structure, think about the issues involved in your design, your hypotheses, and your data. Work with them accordingly.
Go to the next article or see the full series on Easy-to-Confuse Statistical Concepts
When you need to compare a numeric outcome for two groups, what analysis do you think of first? Chances are, it’s the independent samples t-test. But that’s not the only, or always, the best option. In many situations, the Mann-Whitney U test is a better option.
The non-parametric Mann-Whitney U test is also called the Mann-Whitney-Wilcoxon test, or the Wilcoxon rank sum test. Non-parametric means that the hypothesis it’s testing is not about the parameter of a particular distribution.
It is part of a subgroup of non-parametric tests that are rank based. That means that the specific values of the outcomes are not important, only their order. In other words, we will be ranking the outcomes.
Like the t-test, this analysis tests whether two independent groups have similar typical outcomes. You can use it with numeric data, but unlike the t-test, it also works with ordinal data. Like the t-test, it is designed for comparisons, and not for estimation or prediction.
The biggest difference from the t-test is that it does not compare means. The Mann-Whitney U test determines whether a random observation from one group tends to be higher (or lower) than a random observation from the other group. Imagine choosing two observations, one from each group, over and over again. This test will determine whether one group is more likely to have the higher values.
It has many advantages: It is a straightforward comparison of means. There are versions for similar and different variances in the two groups. Many people are familiar with it.
(more…)
One of the important issues with missing data is the missing data mechanism. You may have heard of these: Missing Completely at Random (MCAR), Missing at Random (MAR), and Missing Not at Random (MNAR).
The mechanism is important because it affects how much the missing data bias your results. This has a big impact on what is a reasonable approach to dealing with the missing data. So you have to take it into account in choosing an approach.
The concepts of these mechanisms can be a bit abstract.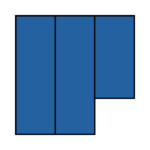
And to top it off, two of these mechanisms have really confusing names: Missing Completely at Random and Missing at Random.
Missing Completely at Random (MCAR)
Missing Completely at Random is pretty straightforward. What it means is what is (more…)