How should I build my model?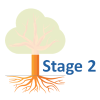
I get this question a lot, and it’s difficult to answer at first glance–it depends too much on your particular situation.
There are really three parts to the approach to building a model: the strategy, the technique to implement that strategy, and the decision criteria used within the technique. (more…)
Repeated measures ANOVA is the approach most of us learned in stats classes for repeated measures and longitudinal data. It works very well in certain designs.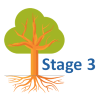
But it’s limited in what it can do. Sometimes trying to fit a data set into a repeated measures ANOVA requires too much data gymnastics. (more…)
Not too long ago, a client asked for help with using Spotlight Analysis to interpret an interaction in a regression model.
Spotlight Analysis? I had never heard of it.
As it turns out, it’s a (snazzy) new name for an old way of interpreting an interaction between a continuous and a categorical grouping variable in a regression model. (more…)
Whenever you run multiple statistical tests on the same set of data, you run into the problem of the Familywise Error Rate. What this means is that the true probability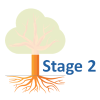
of a type 1 error somewhere in the family of tests you’re running is actually higher than the alpha=.05 you’re using for any given test.
This is a complicated and controversial issue in statistics — even statisticians argue about whether it’s a problem, when it’s a problem, and what to do about it.
In this webinar, we’ll talk about the meaning and consequences of these issues so you can make informed decisions in your data analysis.
We’ll also go through possible solutions, including post-hoc tests and the false discovery rate.
Note: This training is an exclusive benefit to members of the Statistically Speaking Membership Program and part of the Stat’s Amore Trainings Series. Each Stat’s Amore Training is approximately 90 minutes long.
About the Instructor
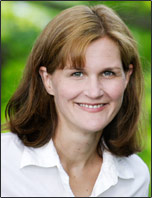
Karen Grace-Martin helps statistics practitioners gain an intuitive understanding of how statistics is applied to real data in research studies.
She has guided and trained researchers through their statistical analysis for over 15 years as a statistical consultant at Cornell University and through The Analysis Factor. She has master’s degrees in both applied statistics and social psychology and is an expert in SPSS and SAS.
Not a Member Yet?
It’s never too early to set yourself up for successful analysis with support and training from expert statisticians.
Just head over and sign up for Statistically Speaking.
You'll get access to this training webinar, 130+ other stats trainings, a pathway to work through the trainings that you need — plus the expert guidance you need to build statistical skill with live Q&A sessions and an ask-a-mentor forum.
One of the most confusing things about statistical analysis is the different vocabulary used for the same, or nearly-but-not-quite-the-same, concepts.
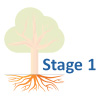
Sometimes this happens just because the same analysis was developed separately within different fields and named twice.
So people in different fields use different terms for the same statistical concept. Try to collaborate with a colleague in a different field and you may find yourself awed by the crazy statistics they’re insisting on.
Other times, there is a level of detail that is implied by one term that isn’t true of the wider, more generic term. This level of detail is often about how the role of variables or effects affects the interpretation of output. (more…)
In this follow-up to December’s webinar, we’ll finish up our discussion of interactions.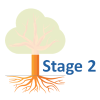
There is something about interactions that is incredibly confusing.
An interaction between two predictor variables means that one predictor variable affects a third variable differently at different values of the other predictor.
How you understand that interaction depends on many things, including:
- Whether one, or both, of the predictor variables is categorical or numerical
- How each of those variables is coded (specifically, whether each categorical variable is dummy or effect coded and whether numerical variables are centered)
- Whether it’s a two-way or three-way interaction
- Whether there is a directionality to the interaction (moderation) or not
Sometimes you need to get pretty sophisticated in your coding, in the output you ask for, and in writing out regression equations.
In this webinar, we’ll examine how to put together and break apart output to understand what your interaction is telling you.
Note: This training is an exclusive benefit to members of the Statistically Speaking Membership Program and part of the Stat’s Amore Trainings Series. Each Stat’s Amore Training is approximately 90 minutes long.
About the Instructor
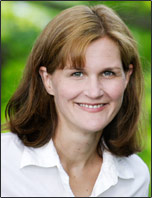
Karen Grace-Martin helps statistics practitioners gain an intuitive understanding of how statistics is applied to real data in research studies.
She has guided and trained researchers through their statistical analysis for over 15 years as a statistical consultant at Cornell University and through The Analysis Factor. She has master’s degrees in both applied statistics and social psychology and is an expert in SPSS and SAS.
Not a Member Yet?
It’s never too early to set yourself up for successful analysis with support and training from expert statisticians.
Just head over and sign up for Statistically Speaking.
You'll get access to this training webinar, 130+ other stats trainings, a pathway to work through the trainings that you need — plus the expert guidance you need to build statistical skill with live Q&A sessions and an ask-a-mentor forum.