Centering a covariate –a continuous predictor variable–can make regression coefficients much more interpretable. That’s a big advantage, particularly when you have many coefficients to interpret. Or when you’ve included terms that are tricky to interpret, like interactions or quadratic terms.
For example, say you had one categorical predictor with 4 categories and one continuous covariate, plus an interaction between them.
First, you’ll notice that if you center your covariate at the mean, there is (more…)
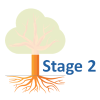
Statistical contrasts are a tool for testing specific hypotheses and model effects, particularly comparing specific group means.
(more…)
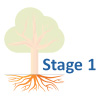
Before you can write a data analysis plan, you have to choose the best statistical test or model. You have to integrate a lot of information about your research question, your design, your variables, and the data itself.
(more…)
A key part of the output in any linear model is the ANOVA table. It has many names in different software procedures, but every regression or ANOVA
model has a table with Sums of Squares, degrees of freedom, mean squares, and F tests. Many of us were trained to skip over this table, but
(more…)
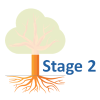
In your statistics class, your professor made a big deal about unequal sample sizes in one-way Analysis of Variance (ANOVA) for two reasons.
1. Because she was making you calculate everything by hand. Sums of squares require a different formula* if sample sizes are unequal, but statistical software will automatically use the right formula. So we’re not too concerned. We’re definitely using software.
2. Nice properties in ANOVA such as the Grand Mean being the intercept in an effect-coded regression model don’t hold when data are unbalanced. Instead of the grand mean, you need to use a weighted mean. That’s not a big deal if you’re aware of it. (more…)
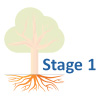
Missing data causes a lot of problems in data analysis. Unfortunately, some of the “solutions” for missing data cause more problems than they solve.
(more…)