One of the tricky parts about dummy coded (0/1) variables is keeping track of what’s a 0 and what’s a 1.
This is made particularly tricky because sometimes your software switches them on you.
Here’s one example in a question I received recently. The context was a Linear Mixed Model, but this can happen in other procedures as well.
I dummy code my categorical variables “0” or “1” but for some reason in the (more…)
Model Building–choosing predictors–is one of those skills in statistics that is difficult to teach. It’s hard to lay out the steps, because at each step, you have to evaluate the situation and make decisions on the next step.
If you’re running purely predictive models, and the relationships among the variables aren’t the focus, it’s much easier. Go ahead and run a stepwise regression model. Let the data give you the best prediction.
But if the point is to answer a research question that describes relationships, you’re going to have to get your hands dirty.
It’s easy to say “use theory” or “test your research question” but that ignores a lot of practical issues. Like the fact that you may have 10 different variables that all measure the same theoretical construct, and it’s not clear which one to use. (more…)
There are three main ways you can approach analyzing repeated measures data, assuming the dependent variable is measured
continuously: repeated measures ANOVA, Mixed Models, and Marginal Models. Let’s take a look at how the three approaches differ and some of their advantages and disadvantages.
For a few, very specific designs, you can get the exact same results from all three approaches. This makes it difficult to figure out what each one is doing, and how to apply them to OTHER designs.
For the sake of the current discussion, I will define repeated measures data as repeated measurements of the same outcome variable on the same individual. The individual is often a person, but could just as easily be a plant, animal, colony, company, etc. For simplicity, I’ll use “individual.” (more…)
If you’re in a field that uses Analysis of Variance, you have surely heard that p-values don’t indicate the size of an effect. You also need to
report effect size statistics.
Why? Because with a big enough sample size, any difference in means, no matter how small, can be statistically significant. P-values are designed to tell you if your result is a fluke, not if it’s big.
Unstandardized Effect Size Statistics
Truly the simplest and most straightforward effect size measure is the difference between two means. And you’re probably already reporting that. But the limitation of this measure as an effect size is not inaccuracy. It’s just hard to evaluate.
If you’re familiar with an area of research and the variables used in that area, you should know if a 3-point difference is big or small, although your readers may not. And if you’re evaluating a new type of variable, it can be hard to tell.
Standardized Effect Size Statistics
Standardized effect size statistics are designed for easier evaluation. They remove the units of measurement, so you don’t have to be familiar with the scaling of the variables.
Cohen’s d is a good example of a standardized effect size measurement. It’s equivalent in many ways to a standardized regression coefficient (labeled beta in some software). Both are standardized measures. They divide the size of the effect by the relevant standard deviations. So instead of being in terms of the original units of X and Y, both Cohen’s d and standardized regression coefficients are in terms of standard deviations.
There are some nice properties of standardized effect size measures. The foremost is you can compare them across variables. And in many situations, seeing differences in terms of number of standard deviations is very helpful.
Limitations
But they are most useful if you can also recognize their limitations. Unlike correlation coefficients, both Cohen’s d and beta can be greater than one. So while you can compare them to each other, you can’t just look at one and tell right away what is big or small. You’re just looking at the effect of the independent variable in terms of standard deviations.
This is especially important to note for Cohen’s d, because in his original book, he specified certain d values as indicating small, medium, and large effects in behavioral research. While the statistic itself is a good one, you should take these size recommendations with a grain of salt (or maybe a very large bowl of salt). What is a large or small effect is highly dependent on your specific field of study, and even a small effect can be theoretically meaningful.
Variance Explained
Another set of effect size measures have a more intuitive interpretation, and are easier to evaluate. They include Eta Squared, Partial Eta Squared, and Omega Squared. Like the R Squared statistic, they all have the intuitive interpretation of the proportion of the variance accounted for.
Eta Squared is calculated the same way as R Squared, and has the most equivalent interpretation: out of the total variation in Y, the proportion that can be attributed to a specific X.
Eta Squared, however, is used specifically in ANOVA models. Each effect in the model has its own Eta Squared. So you get a specific, intuitive measure of the effect of that variable.
Eta Squared has two drawbacks, however. One is that as you add more variables to the model, the proportion explained by any one variable will automatically decrease. This makes it hard to compare the effect of a single variable in different studies.
Partial Eta Squared solves this problem, but has a less intuitive interpretation. There, the denominator is not the total variation in Y, but the unexplained variation in Y plus the variation explained just by that X. So any variation explained by other Xs is removed from the denominator. This allows a researcher to compare the effect of the same variable in two different studies, which contain different covariates or other factors.
In a one-way ANOVA, Eta Squared and Partial Eta Squared will be equal. But this isn’t true in models with more than one independent variable.
The drawback for Eta Squared is that it is a biased measure of population variance explained (although it is accurate for the sample). It always overestimates it.
This bias gets very small as sample size increases. For small samples, an unbiased effect size measure is Omega Squared. Omega Squared has the same basic interpretation, but uses unbiased measures of the variance components. Because it is an unbiased estimate of population variances, Omega Squared is always smaller than Eta Squared.
See my post containing equations of all these effect size measures and a list of great references for further reading on effect sizes.
There are many effect size statistics for ANOVA and regression, and as you may have noticed, journal editors are now requiring you include one.
Unfortunately, the one your editor wants or is the one most appropriate to your research may not be the one your software makes available (SPSS, for example, reports Partial Eta Squared only, although it labels it Eta Squared in early versions).
Luckily, all the effect size measures are relatively easy to calculate from information in the ANOVA table on your output. Here are a few common ones: (more…)
If you’ve compared two textbooks on linear models, chances are, you’ve seen two different lists of assumptions.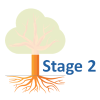
I’ve spent a lot of time trying to get to the bottom of this, and I think it comes down to a few things.
1. There are four assumptions that are explicitly stated along with the model, and some authors stop there.
2. Some authors are writing for introductory classes, and rightfully so, don’t want to confuse students with too many abstract, and sometimes untestable, (more…)