The ICC, or Intraclass Correlation Coefficient, can be very useful in many statistical situations, but especially so in Linear Mixed Models.
Linear Mixed Models are used when there is some sort of clustering in the data.
Two common examples of clustered data include:
- individuals were sampled within sites (hospitals, companies, community centers, schools, etc.). The site is the cluster.
- repeated measures or longitudinal data where multiple observations are collected from the same individual. The individual is the cluster in which multiple observations are (more…)
I received the following email from a reader after sending out the last article: Opposite Results in Ordinal Logistic Regression—Solving a Statistical Mystery.
And I agreed I’d answer it here in case anyone else was confused.
Karen’s explanations always make the bulb light up in my brain, but not this time.
With either output,
The odds of 1 vs > 1 is exp[-2.635] = 0.07 ie unlikely to be 1, much more likely (14.3x) to be >1
The odds of £2 vs > 2 exp[-0.812] =0.44 ie somewhat unlikely to be £2, more likely (2.3x) to be >2
SAS – using the usual regression equation
If NAES increases by 1 these odds become (more…)
A number of years ago when I was still working in the consulting office at Cornell, someone came in asking for help interpreting their ordinal logistic regression results.
The client was surprised because all the coefficients were backwards from what they expected, and they wanted to make sure they were interpreting them correctly.
It looked like the researcher had done everything correctly, but the results were definitely bizarre. They were using SPSS and the manual wasn’t clarifying anything for me, so I did the logical thing: I ran it in another software program. I wanted to make sure the problem was with interpretation, and not in some strange default or (more…)
One great thing about logistic regression, at least for those of us who are trying to learn how to use it, is that the predictor variables work exactly the same way as they do in linear regression.
Dummy coding, interactions, quadratic terms–they all work the same way.
Dummy Coding
In pretty much every regression procedure in every stat software, the default way to code categorical variables is with dummy coding.
All dummy coding means is recoding the original categorical variable into a set of binary variables that have values of one and zero. You may find it helpful to (more…)
In all linear regression models, the intercept has the same definition: the mean of the response, Y, when all predictors, all X = 0.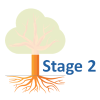
But “when all X=0” has different implications, depending on the scale on which each X is measured and on which terms are included in the model.
So let’s specifically discuss the meaning of the intercept in some common models: (more…)
Recently I gave a webinar The Steps to Running Any Statistical Model. A few hundred people were live on the webinar. We held a Q&A session at the end, but as you can imagine, we didn’t have time to get through all the questions.
This is the first in a series of written answers to some of those questions. I’ve tried to sort them by the step each is about.
A written list of the steps is available here.
If you missed the webinar, you can view the video here. It’s free.
Questions about Step 1. Write out research questions in theoretical and operational terms
Q: In using secondary data research designing, have you found that this type of data source affects the research question? That is, should one have a strong understanding of the data to ensure their theoretical concept can be operational to fit the data? My research question changes the more I learn.
Yes. There’s no point in asking research questions that the data you have available can’t answer.
So the order of the steps would have to change—you may have to start with a vague idea of the type of research question you want to ask, but only refine it after doing some descriptive statistics, or even running an initial model.
Q: How soon in the process should one start with the first group of steps?
You want to at least start thinking about them as you’re doing the lit review and formulating your research questions.
Think about how you could measure variables, which ones are likely to be collinear or have a lot of missing data. Think about the kind of model you’d have to do for each research question.
Think of a scenario where the same research question could be operationalized such that the dependent variable is measured either continuous or ordered categories. An easy example is income in dollars measured by actual income or by income categories.
By all means, if people can answer the question with a real and accurate number, your analysis will be much, much easier. In many situations, they can’t. They won’t know, remember, or tell you their exact income. If so, you may have to use categories to prevent missing data. But these are things to think about early.
Q: where in the process do you use existing lit/results to shape the research question and modeling?
I would start by putting the literature review before Step 1. You’ll use that to decide on a theoretical research question, as well as ways to operationalize it..
But it will help you other places as well. For example, it helps the sample size calculations to have variance estimates from other studies. Other studies may give you an idea of variables that are likely to have missing data, too little variation to include as predictors. They may change your exploratory factor analysis in Step 7 to a confirmatory one.
In fact, just about every step can benefit from a good literature review.
If you missed the webinar, you can view the video here. It’s free.