A central concept in statistics is the level of measurement of a variable. It’s so important to everything you do with data that it’s usually taught within the first week in every intro stats class.
But even something so fundamental can be tricky once you start working with real data. (more…)
One of the most confusing things about statistical analysis is the different vocabulary used for the same, or nearly-but-not-quite-the-same, concepts.
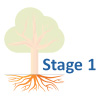
Sometimes this happens just because the same analysis was developed separately within different fields and named twice.
So people in different fields use different terms for the same statistical concept. Try to collaborate with a colleague in a different field and you may find yourself awed by the crazy statistics they’re insisting on.
Other times, there is a level of detail that is implied by one term that isn’t true of the wider, more generic term. This level of detail is often about how the role of variables or effects affects the interpretation of output. (more…)
A normal probability plot is extremely useful for testing normality assumptions. It’s more precise than a histogram, which can’t pick up subtle deviations, and doesn’t suffer from too much or too little power, as do tests of normality.
There are two versions of normal probability plots: Q-Q and P-P. I’ll start with the Q-Q. (more…)
You may have never heard of listwise deletion for missing data, but you’ve probably used it.
Listwise deletion means that any individual in a data set is deleted from an analysis if they’re missing data on any variable in the analysis.
It’s the default in most software packages.
Although the simplicity of it is a major advantage, it causes big problems in many missing data situations.
But not always. If you happen to have one of the uncommon missing data situations in which (more…)
In a statistical model–any statistical model–there is generally one way that a predictor X and a response Y can relate: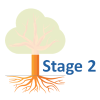

This relationship can take on different forms, of course, like a line or a curve, but there’s really only one relationship here to measure.
Usually the point is to model the predictive ability, the effect, of X on Y.
In other words, there is a clear response variable*, although not necessarily a causal relationship. We could have switched the direction of the arrow to indicate that Y predicts X or used a two-headed arrow to show a correlation, with no direction, but that’s a whole other story.
For our purposes, Y is the response variable and X the predictor.
But a third variable–another predictor–can relate to X and Y in a number of different ways. How this predictor relates to X and Y changes how we interpret the relationship between X and Y. (more…)
Someone recently asked me if they need to learn R. In responding, it struck me that this is another way that learning a stat package is like learning a new language.
The metaphor is extremely helpful for deciding when and how to learn a new stat package, and to keep you going when the going gets rough. (more…)