A common situation with count outcome variables is there are a lot of zero values. The Poisson distribution used for modeling count variables takes into account that zeros are often the most
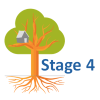
common value, but sometimes there are even more zeros than the Poisson distribution can account for.
This can happen in continuous variables as well–most of the distribution follows a beautiful normal distribution, except for the big stack of zeros.
This webinar will explore two ways of modeling zero-inflated data: the Zero Inflated model and the Hurdle model. Both assume there are two different processes: one that affects the probability of a zero and one that affects the actual values, and both allow different sets of predictors for each process.
We’ll explore these models as well as some related models, like Zero-One Inflated Beta models for proportion data.
Note: This training is an exclusive benefit to members of the Statistically Speaking Membership Program and part of the Stat’s Amore Trainings Series. Each Stat’s Amore Training is approximately 90 minutes long.
(more…)
Proportion and percentage data are tricky to analyze.
Much like count data, they look like they should work in a linear model.
They’re numerical. They’re often continuous.
And sometimes they do work. Some proportion data do look normally distributed so estimates and p-values are reasonable.
But more often they don’t. So estimates and p-values are a mess. Luckily, there are other options. (more…)
Like the chicken and the egg, there’s a question about which comes first: run a model or test assumptions? Unlike the chickens’, the model’s question has an easy answer.
There are two types of assumptions in a statistical model. Some are distributional assumptions about the residuals. Examples include independence, normality, and constant variance in a linear model.
Others are about the form of the model. They include linearity and (more…)
One common reason for running Principal Component Analysis (PCA) or Factor Analysis (FA) is variable reduction.
In other words, you may start with a 10-item scale meant to measure something like Anxiety, which is difficult to accurately measure with a single question.
You could use all 10 items as individual variables in an analysis–perhaps as predictors in a regression model.
But you’d end up with a mess.
Not only would you have trouble interpreting all those coefficients, but you’re likely to have multicollinearity problems.
And most importantly, you’re not interested in the effect of each of those individual 10 items on your (more…)
A few years ago, I was in Syracuse for a family trip to the zoo. Syracuse is about 50 miles from where I live and it has a very nice little zoo.
One year was particularly exciting because a Trader Joe’s just opened in Syracuse. We don’t have one where we live* (sadly!) so we always stock up on our favorite specialty groceries when we’re near a Trader Joe’s.
On this particular trip, though, we had an unwelcome surprise. My credit card card company believed my Trader Joe’s spree was fraudulent and declined the transaction. I got a notice on my phone and was able to fix it right away, so it wasn’t the big inconvenience it could have been.
But this led us to wonder what it was about the transaction that led the bank to believe it was fraudulent. Do credit card thieves often skip town and go grocery shopping?
The bank was clearly betting so. It must have a statistical model for aspects of a transaction that are likely enough to be fraudulent that it shuts it down. (more…)
Effect size statistics are expected by many journal editors these days.
If you’re running an ANOVA, t-test, or linear regression model, it’s pretty straightforward which ones to report.
Things get trickier, though, once you venture into other types of models.
(more…)