R² is such a lovely statistic, isn’t it? Unlike so many of the others, it makes sense–the percentage of variance in Y accounted for by a model.
I mean, you can actually understand that. So can your grandmother. And the clinical audience you’re writing the report for.
A big R² is always good and a small one is always bad, right?
Well, maybe. (more…)
If you learned much about calculating power or sample sizes in your statistics classes, chances are, it was on something very, very simple, like a z-test.
But there are many design issues that affect power in a study that go way beyond a z-test. Like:
- repeated measures
- clustering of individuals
- blocking
- including covariates in a model
Regular sample size software can accommodate some of these issues, but not all. And there is just something wonderful about finding a tool that does just what you need it to.
Especially when it’s free.
Enter Optimal Design Plus Empirical Evidence software. (more…)
Generalized linear models, linear mixed models, generalized linear mixed models, marginal models, GEE models. You’ve probably heard of more than one of them and you’ve probably also heard that each one is an extension of our old friend, the general linear model.
This is true, and they extend our old friend in different ways, particularly in regard to the measurement level of the dependent variable and the independence of the measurements. So while the names are similar (and confusing), the distinctions are important.
It’s important to note here that I am glossing over many, many details in order to give you a basic overview of some important distinctions. These are complicated models, but I hope this overview gives you a starting place from which to explore more. (more…)
You may have noticed conflicting advice about whether to leave insignificant effects in a model or take them out in order to simplify the model.
One effect of leaving in insignificant predictors is on p-values–they use up precious df in small samples. But if your sample isn’t small, the effect is negligible.
The bigger effect is on interpretation, and really the above cases are about whether it aids interpretation to leave them in. Models do get so cluttered it’s hard to figure out what’s going on, and it makes sense to eliminate effects that aren’t serving a purpose, but even insignificant effects can have a purpose. (more…)
It’s really easy to mix up the concepts of association (as measured by correlation) and interaction. Or to assume if two variables interact, they must be associated. But it’s not actually true.
In statistics, they have different implications for the relationships among your variables. This is especially true when the variables you’re talking about are predictors in a regression or ANOVA model.
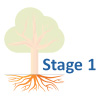
Association
Association between two variables means the values of one variable relate in some way to the values of the other. It is usually measured by correlation for two continuous variables and by cross tabulation and a Chi-square test for two categorical variables.
Unfortunately, there is no nice, descriptive measure for association between one (more…)
Last month I did a webinar on Poisson and negative binomial models for count data. With a few hundred participants, we ran out of time to get through all the questions, so I’m answering some of them here on the blog.
This set of questions are all related to when it’s appropriate to treat count data as continuous and run the more familiar and simpler linear model.
Q: Do you have any guidelines or rules of thumb as far as how many discrete values an outcome variable can take on before it makes more sense to just treat it as continuous?
The issue usually isn’t a matter of how many values there are.
(more…)