One area in statistics where I see conflicting advice is how to analyze pre-post data. I’ve seen this myself in consulting. A few years ago, I received a call from a distressed client. Let’s call her Nancy.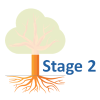
Nancy had asked for advice about how to run a repeated measures analysis. The advisor told Nancy that actually, a repeated measures analysis was inappropriate for her data.
Nancy was sure repeated measures was appropriate. This advice led her to fear that she had grossly misunderstood a very basic tenet in her statistical training.
The Study Design
Nancy had measured a response variable at two time points for two groups. The intervention group received a treatment and a control group did not. Participants were randomly assigned to one of the two groups.
The researcher measured each participant before and after the intervention.
Analyzing the Pre-Post Data
Nancy was sure that this was a classic repeated measures experiment. It has (more…)
I don’t usually get into discussions here about the teaching of statistics, as that’s not really the point of this blog.
Even so, I found this idea fascinating, and since this blog is about learning statistics, I thought you may find it interesting as well.
The following is a TED talk by Arthur Benjamin, who is a math professor at Harvey Mudd College. Let me start by saying he is awesome. I already watched his Mathemagician TED talk with my kids*, so when I found this, I already expected it to be very good.
I wasn’t disappointed.
*if you too are on an active campaign to instill a love of math in your kids, I highly recommend it.
Here are two of my favorite quotes, keeping in mind that I took calculus in high school and I LOVED it. Even so, he’s got some really good points:
“Very few people actually use calculus in a conscious, meaningful way in their day-to-day lives. On the other hand, statistics–that’s a subject that you could, and should, use on a daily basis.”
“If it’s taught properly, it can be a lot of FUN. I mean, probability and statistics–it’s the mathematics of games and gambling, it’s…it’s analyzing trends, it’s predicting the future.”
If you find the video isn’t working, you can watch it directly on the TED site (this is the first time I’ve embedded a video). 🙂
If you’ve ever worked with multilevel models, you know that they are an extension of linear models. For a researcher learning them, this is both good and bad news.
The good side is that many of the concepts, calculations, and results are familiar. The down side of the extension is that everything is more complicated in multilevel models.
This includes power and sample size calculations. (more…)
The first real data set I ever analyzed was from my senior honors thesis as an undergraduate psychology major. I had taken both intro stats and an ANOVA class, and I applied all my new skills with gusto, analyzing every which way.
It wasn’t too many years into graduate school that I realized that these data analyses were a bit haphazard and not at all well thought out. 20 years of data analysis experience later and I realized that’s just a symptom of being an inexperienced data analyst.
But even experienced data analysts can get off track, especially with large data sets with many variables. It’s just so easy to try one thing, then another, and pretty soon you’ve spent weeks getting nowhere.
(more…)
Standardized regression coefficients remove the unit of measurement of predictor and outcome variables. They are sometimes called betas, but I don’t like to use that term because there are too many other, and too many related, concepts that are also called beta.
There are many good reasons to report them:
- They serve as standardized effect size statistics.
- They allow you to compare the relative effects of predictors measured on different scales.
- They make journal editors and committee members happy in fields where they are commonly reported. (more…)
I received an e-mail from a researcher in Canada that asked about communicating logistic regression results to non-researchers. It was an important question, and there are a number of parts to it.
With the asker’s permission, I am going to address it here.
To give you the full context, she explained in a follow-up email that she is communicating to a clinical audience who will be using the results to make clinical decisions. They need to understand the size of an effect that an intervention will provide. She refers to an output I presented in my webinar on Probability, Odds, and Odds Ratios, which you can view free here.
Question:
I just went through the two lectures re: logistic regression and prob/odds/odds ratios. I completely understand (more…)