One of the many decisions you have to make when model building is which form each predictor variable should take. One specific version of this
decision is whether to combine categories of a categorical predictor.
The greater the number of parameter estimates in a model the greater the number of observations that are needed to keep power constant. The parameter estimates in a linear (more…)
Learning how to analyze data can be frustrating at times. Why do statistical software companies have to add to our confusion?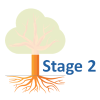
I do not have a good answer to that question. What I will do is show examples. In upcoming blog posts, I will explain what each output means and how they are used in a model.
We will focus on ANOVA and linear regression models using SPSS and Stata software. As you will see, the biggest differences are not across software, but across procedures in the same software.
(more…)
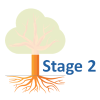
Our analysis of linear regression focuses on parameter estimates, z-scores, p-values and confidence levels. Rarely in regression do we see a discussion of the estimates and F statistics given in the ANOVA table above the coefficients and p-values.
And yet, they tell you a lot about your model and your data. Understanding the parts of the table and what they tell you is important for anyone running any regression or ANOVA model.
(more…)
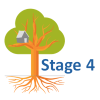
The last, and sometimes hardest, step for running any statistical model is writing up results.
As with most other steps, this one is a bit more complicated for structural equation models than it is for simpler models like linear regression.
Any good statistical report includes enough information that someone else could replicate your results with your data.
(more…)
Any time you report estimates of parameters in a statistical analysis, it’s important to include their confidence intervals.
How confident are you that you can explain what they mean? Even those of us who have a solid understand of confidence intervals get tripped up by the wording.
The Wording for Describing Confidence Intervals
Let’s look at an example. (more…)
There are a number of simplistic methods available for tackling the problem of missing data. Unfortunately there is a very high likelihood that each of these simplistic methods introduces bias into our model results.
Multiple imputation is considered to be the superior method of working with missing data. It eliminates the bias introduced by the simplistic methods in many missing data situations.
(more…)