by Maike Rahn, PhD
In previous posts in this series, we discussed factors and factor loadings and rotations. In this post, I would like to address another important detail for a successful factor analysis, the type of variables that you include in your analysis.
What type of variable?
Ideally, factor analysis is conducted with continuous variables that are normally distributed since factor analysis is based on a correlation matrix.
However, you will undoubtedly find many factor analyses that include ordinal variables, particularly Likert scale items.
While technically, Likert items don’t meet the assumptions of Factor Analysis, at least in some situations the results have been found to be quite reasonable. For example, Lubke & Muthen, (2004) found that Confirmatory Factor Analysis on a single homogenous group worked, as long as items have at least seven values.
Some researchers include variables with fewer than seven values into their factor analysis. Sometimes this cannot be avoided, if you are using an already published scale.
Last, there is an interesting discussion about including binary variables in a factor analysis in the Sage Publications booklet “Factor analysis. Statistical methods and practical issues” (Kim and Mueller, 1978; page 75).
Correct coding of variables
It is important to prepare your variables in advance. For example, if you anticipate finding a socioeconomic factor, create your ordinal variable occupation with levels from lowest to highest to make sure that you have a positive factor loading with your factor.
Occupational categories |
Levels of occupation variable |
Nurse’s Aid |
1 |
Administrative assistant |
2 |
Nurse |
3 |
Nurse manager |
4 |
Physician |
5 |
Department chair |
6 |
Director |
7 |
The reason for this preparation is that you will wind up with factor solutions that are easily interpretable, because variables that are coded in the same direction as the factor will always have a positive factor loading. On the other hand, variables that have an inverse association with the factor will always have a negative factor loading.
Kim, Jae-On and Mueller, Charles W (1978) Factor analysis. Statistical methods and practical issues. Series: Quantitative Applications in the Social Sciences. Sage Publications: Beverly Hills, CA.
Complex Surveys use a sampling technique other than a simple random sample. Terms you may have heard in this area include cluster sampling, stratified sampling, oversampling, two-stage
sampling, and primary sampling unit.
Complex Samples require statistical methods that take the exact sampling design into account to ensure accurate results.
In this webinar, guest instructor Dr. Trent Buskirk will give you an overview of the common sampling techniques and their effects on data analysis.
Note: This training is an exclusive benefit to members of the Statistically Speaking Membership Program and part of the Stat’s Amore Trainings Series. Each Stat’s Amore Training is approximately 90 minutes long.
About the Instructor
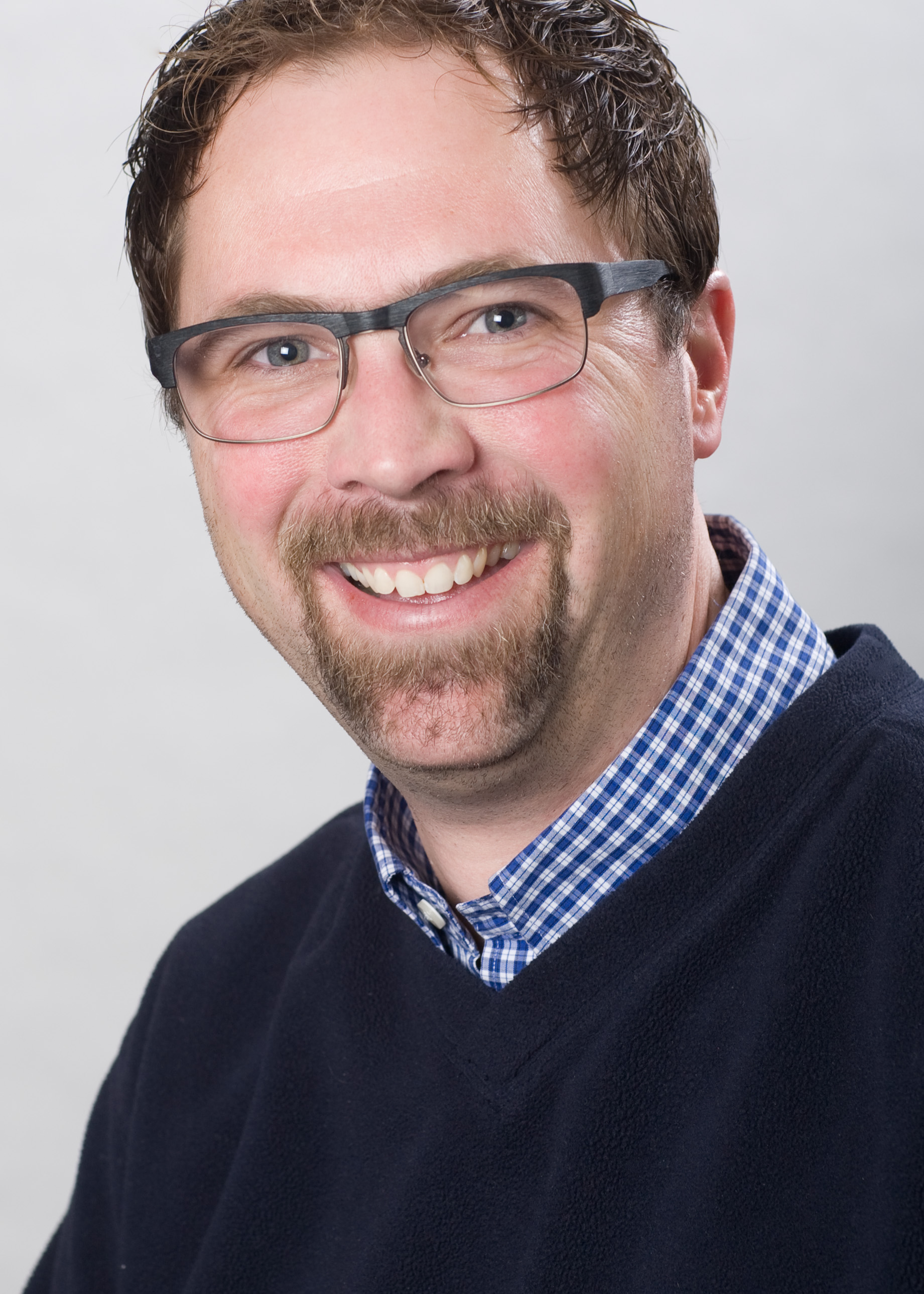
Trent D. Buskirk, Ph.D. is the Vice President of Statistics and Methodology, Marketing Systems Group.
Dr. Buskirk has more than 15 years of professional and academic experience in the fields of survey research, statistics, as well as SPSS, SAS, and R.
Dr. Buskirk has taught for more than a decade at the University of Nebraska and Saint Louis University where he was an Associate Professor of Biostatistics in the School of Public Health.
Not a Member Yet?
It’s never too early to set yourself up for successful analysis with support and training from expert statisticians.
Just head over and sign up for Statistically Speaking.
You'll get access to this training webinar, 130+ other stats trainings, a pathway to work through the trainings that you need — plus the expert guidance you need to build statistical skill with live Q&A sessions and an ask-a-mentor forum.
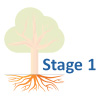
In Part 6, let’s look at basic plotting in R. Try entering the following three commands together (the semi-colon allows you to place several commands on the same line).
x <- seq(-4, 4, 0.2) ; y <- 2*x^2 + 4*x - 7
plot(x, y)
(more…)
by Maike Rahn, PhD
In the previous blogs I wrote about the basics of running a factor analysis. Real-life factor analysis can become complicated. Here are some of the more common problems researchers encounter and some possible solutions:
- The factor loadings in your confirmatory factor analysis are only |0.5| or less.
Solution: lower the cut-offs of your factor loadings, provided that lower factor loadings are expected and accepted in your field.
- Your confirmatory factor analysis does not show the hypothesized number of factors.
Solution 1: you were not able to validate the factor structure in your sample; your analysis with this sample did not work out.
Solution 2: your factor analysis has just become exploratory. Something is going on with your sample that is different from the samples used in other studies. Find out what it is.
- A few key variables in your confirmatory factor analysis do not behave as expected and/or are correlated with the wrong factor.
Solution: the good news is that you found the hypothesized factors. The bad news is (more…)
by Maike Rahn, PhD
When are factor loadings not strong enough?
Once you run a factor analysis and think you have some usable results, it’s time to eliminate variables that are not “strong” enough. They are usually the ones with low factor loadings, although additional criteria should be considered before taking out a variable.
As a rule of thumb, your variable should have a rotated factor loading of at least |0.4| (meaning ≥ +.4 or ≤ –.4) onto one of the factors in order to be considered important. (more…)
by Maike Rahn, PhD
One of the hardest things to determine when conducting a factor analysis is how many factors to settle on. Statistical programs provide a number of criteria to help with the selection.
Eigenvalue > 1
Programs usually have a default cut-off for the number of generated factors, such as all factors with an eigenvalue of ≥1.
This is because a factor with an eigenvalue of 1 accounts for as much variance as a single variable, and the logic is that only factors that explain at least the same amount of variance as a single variable is worth keeping.
But often a cut-off of 1 results in more factors than the user bargained for or (more…)